Advancing Precision in Medical Diagnostics using AI Expert-Guided Transformers for Enhanced Accuracy
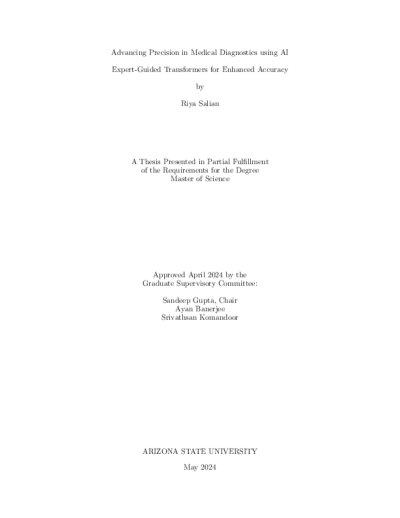
Description
In the realm of medical diagnostics, achieving heightened accuracy is paramount, leading to the meticulous refinement of AI Models through expert-guided tuning aiming to bolster the precision by ensuring their adaptability to complex datasets and optimizing outcomes across various healthcare sectors. By incorporating expert knowledge into the fine-tuning process, these advanced models become proficient at navigating the intricacies of medical data, resulting in more precise and dependable diagnostic predictions. As healthcare practitioners grapple with challenges presented by conditions requiring heightened sensitivity, such as cardiovascular diseases, continuous blood glucose monitoring, the application of nuanced refinement in Transformer Models becomes indispensable. Temporal data, a common feature in medical diagnostics, presents unique challenges for Transformer Models characterized by sequential observations over time, requiring models to capture intricate temporal dependencies and complex patterns effectively. In the study, two pivotal healthcare scenarios are delved into: the detection of Coronary Artery Disease (CAD) using Stress ECGs and the identification of psychological stress using Continuous Glucose Monitoring (CGM) data. The CAD dataset was obtained from the Mayo Clinic Integrated Stress Center (MISC) database, which encompassed 100,000 Exercise Stress ECG signals (n=1200), sourced from multiple Mayo Clinic facilities. For the CGM scenario, expert knowledge was utilized to generate synthetic data using the Bergman minimal model, which was then fed to the transformers for classification. Implementation in the CAD example yielded a remarkable 28% Positive Predictive Value (PPV) improvement over the current state-of-the-art, reaching an impressive 91.2%. This significant enhancement demonstrates the efficacy of the approach in enhancing diagnostic accuracy and underscores the transformative impact of expert-guided fine-tuning in medical diagnostics.
Date Created
The date the item was original created (prior to any relationship with the ASU Digital Repositories.)
2024
Agent
- Author (aut): Salian, Riya
- Thesis advisor (ths): Gupta, Sandeep
- Committee member: Banerjee, Ayan
- Committee member: Komandoor, Srivathsan
- Publisher (pbl): Arizona State University