Frameworks of Many into the Creation of One
Name
Frameworks of Many into the Creation of One
Document
MIME type
application/pdf
File size
2605699
Media Use
Original Name
Housworth_asu_0010N_24114.pdf
Document
Document
An Evaluation Study of Object Interaction Framework Design for XR-Enabled Games
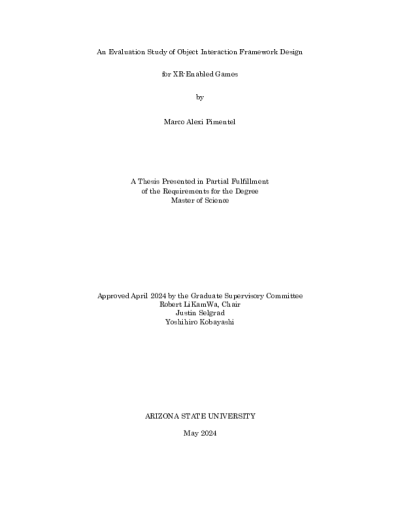
Description
In game development, interaction frameworks provide generic functionality for users to engage with virtual worlds and are required to enable this on nonstandard hardware such as extended reality platforms. Currently, no publicly accessible frameworks exist that implement interactive world objects in XR settings, prompting the question: if one were to be made, how and why would it be usable? This thesis explores the properties that make an XR-enabled object interaction
framework usable by game developers and game designers. This thesis introduces the basic form of such a framework and the design of a set of user studies centered around this framework’s utilization in a game development workflow. User feedback is gathered for the study’s results, and is evaluated around user perception of framework expressiveness, extensibility, and ease of use. The results of the study found that users primarily judged usability through comparisons to real-world equivalents, utilization of conventional systems, object interactivity, clarity of framework components, usability of framework toolkits and these are discussed in relation to existing research on virtual object interaction.
Date Created
The date the item was original created (prior to any relationship with the ASU Digital Repositories.)
2024
Agent
- Author (aut): Pimentel, Marco Alexi
- Thesis advisor (ths): LiKamWa, Robert R.L.
- Committee member: Selgrad, Justin J.S.
- Committee member: Kobayashi, Yoshihiro Y.K.
- Publisher (pbl): Arizona State University
Somatic Symptoms in Mexican-Origin Children: The Role of Familism and Family Conflict in the Context of COVID-19
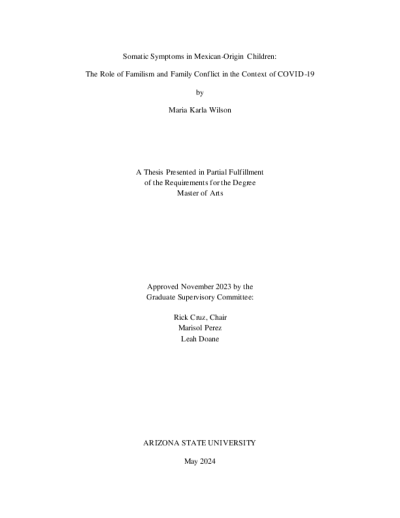
Description
Mexican-origin families (MO) have been more negatively impacted by the COVID-19 pandemic compared to White European-Americans. Latinx youth also reported increased concern about somatic symptoms (i.e. bodily symptoms) during the pandemic compared to non-Latinx peers. Current research on the pandemic indicates that cohesive and supportive families fare better than families within high-conflict households. However, no research has directly examined parent-child conflict during the pandemic in relation to youth somatic symptoms. Previous studies considered cultural proxies as predictors of somatic symptoms in Latinx children, the specific influence of prominent cultural values like familism remains less explored. Familism, emphasizing family well-being, is considered protective for Latinx youth, but evidence suggests it may pose risks in high parent-child conflict households. Utilizing Qualtrics panel, I collected data from 301 MO parents reporting on a target child (Mage= 11.4 years, SD= 3.7; 50.2% female) between March and June 2022. Approximately 40% of parents completed the survey in Spanish. Parental familism dimensions (support, family as referent, and obligations) were assessed as well as youth somatic symptoms. Changes in parent-child conflict and changes in social and family contacts due to the pandemic were also measured. Logistic regression models revealed that greater increases in conflict significantly predicted the presence of youth somatic symptoms (OR = 0.52, 95% CI= [0.27, 1.00]. Unexpectedly, total familism did not significantly moderate the relation between change in parent-child conflict and the presence of somatic symptoms (RR = 1.16, 95% CI= [0.99, 1.36]. However, post hoc analyses revealed that parental familism support was the only dimension of familism that was directly associated with the count of child somatic symptoms (RR= 0.81, 95% Confidence Interval [CI]= 0.71, 0.93). Parental familism as a referent significantly moderated the relation between change in parent-child conflict and the count of somatic symptoms (RR= 1.19, 95% CI = [1.01, 1.41]), such that higher familism values strengthened the positive relationship of change in parent-child conflict and higher count of somatic symptoms. Findings emphasize the need for future longitudinal research, considering medical conditions, to understand how parental familism values, particularly support, may buffer against somatic symptoms for Latinx youth.
Date Created
The date the item was original created (prior to any relationship with the ASU Digital Repositories.)
2024
Agent
- Author (aut): Wilson, Maria Karla
- Thesis advisor (ths): Cruz, Rick A.
- Committee member: Perez, Marisol
- Committee member: Doane, Leah
- Publisher (pbl): Arizona State University
Adaptation for whom? A Study of the Conceptualization and Experience of Planned Adaptation Interventions in India
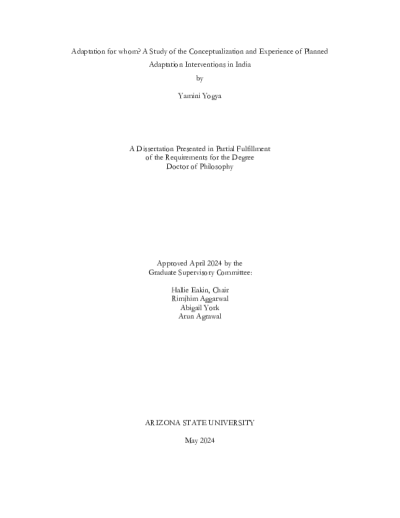
Description
Adaptation to climate change is a core sustainability challenge across the Global South. Development and government organizations conceptualize and govern climate adaptation by creating national and sub-national action plans and implementing projects. This dissertation confronts the inherent tensions that arise when formal planned adaptation interventions encounter the complex, often messy realities of the implementation context. In doing so, this research examines how planned adaptation—with its incentives, provisioned resources, prescribed behaviors, and expectations of commitment from target beneficiaries —interacts with individuals and communities already balancing diverse risks while pursuing their livelihood aspirations. Two broad questions guide this dissertation: 1) how is adaptation envisioned by planners and practitioners? and, 2) how do project beneficiaries engage with, and experience planned adaptation interventions? The research employs an exploratory and inductive qualitative research design. Using Foucault’s lens of governmentality, this research utilises document analysis to examine how the first wave of Indian adaptation projects envision goals, conceptualize problems, delineate roles, and frame expectations of intended beneficiaries. Next, using a case study of an adaptation project implemented in Uttarakhand, India, the study examines the motivations and associated trade-offs behind the engagement and disengagement of the intended beneficiaries: smallholder farmers. Insights from gender-differentiated focus group discussions guide this analysis. Both inquiries are supplemented with findings from semi-structured interviews with Indian adaptation experts and project implementers. The analysis finds that: 1) project reports construct identities of the climate vulnerable beneficiary, implicitly assigning roles and transferring responsibilities for sustaining adaptation efforts beyond project timelines, 2) project participants are not default beneficiaries, but instead exercise agency in decision-making by either opting-in or opting-out of planned initiatives, and 3) the implicit and explicit costs of engaging in planned adaptation interventions are substantial, encompassing significant contributions of time, physical labor, and active participation during and post the project period. This dissertation challenges existing notions of whom planned adaptation serves, and to what end, offering new insights into its design and effectiveness. Furthermore, this research suggests that for planned adaptation to be sustainable, a concerted effort to align with evolving needs, aspirations and livelihood shifts of those on the frontlines of climate change is essential.
Date Created
The date the item was original created (prior to any relationship with the ASU Digital Repositories.)
2024
Agent
- Author (aut): Yogya, Yamini
- Thesis advisor (ths): Eakin, Hallie
- Committee member: Aggarwal, Rimjhim
- Committee member: York, Abigail
- Committee member: Agrawal, Arun
- Publisher (pbl): Arizona State University
Making the Best of What We Have: Novel Strategies for Training Neural Networks under Restricted Labeling Information
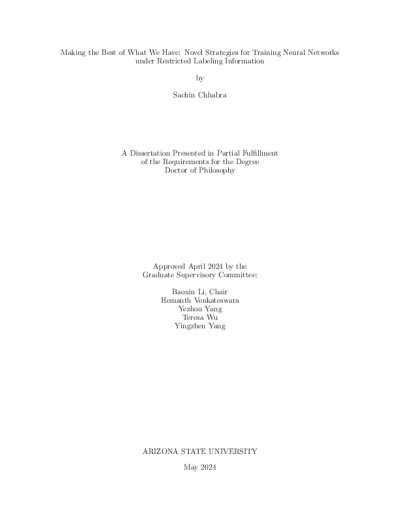
Description
Recent advancements in computer vision models have largely been driven by supervised training on labeled data. However, the process of labeling datasets remains both costly and time-intensive. This dissertation delves into enhancing the performance of deep neural networks when faced with limited or no labeling information. I address this challenge through four primary methodologies: domain adaptation, self-supervision, input regularization, and label regularization. In situations where labeled data is unavailable but a similar dataset exists, domain adaptation emerges as a valuable strategy for transferring knowledge from the labeled dataset to the target dataset. This dissertation introduces three innovative domain adaptation methods that operate at pixel, feature, and output levels.Another approach to tackle the absence of labels involves a novel self-supervision technique tailored to train Vision Transformers in extracting rich features.
The third and fourth approaches focus on scenarios where only a limited amount of labeled data is available. In such cases, I present novel regularization techniques designed to mitigate overfitting by modifying the input data and the target labels, respectively.
Date Created
The date the item was original created (prior to any relationship with the ASU Digital Repositories.)
2024
Agent
- Author (aut): Chhabra, Sachin
- Thesis advisor (ths): Li, Baoxin
- Committee member: Venkateswara, Hemanth
- Committee member: Yang, Yezhou
- Committee member: Wu, Teresa
- Committee member: Yang, Yingzhen
- Publisher (pbl): Arizona State University
Multi-Camera Bird-Eye-View Occupancy Detection for Intelligent Transportation System
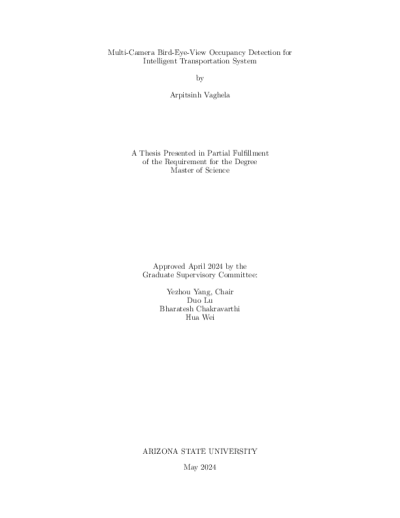
Description
3D perception poses a significant challenge in Intelligent Transportation Systems (ITS) due to occlusion and limited field of view. The necessity for real-time processing and alignment with existing traffic infrastructure compounds these limitations. To counter these issues, this work introduces a novel multi-camera Bird-Eye View (BEV) occupancy detection framework. This approach leverages multi-camera setups to overcome occlusion and field-of-view limitations while employing BEV occupancy to simplify the 3D perception task, ensuring critical information is retained. A noble dataset for BEV Occupancy detection, encompassing diverse scenes and varying camera configurations, was created using the CARLA simulator. Subsequent extensive evaluation of various Multiview occupancy detection models showcased the critical roles of scene diversity and occupancy grid resolution in enhancing model performance. A structured framework that complements the generated data is proposed for data collection in the real world. The trained model is validated against real-world conditions to ensure its practical application, demonstrating the influence of robust dataset design in refining ITS perception systems. This contributes to significant advancements in traffic management, safety, and operational efficiency.
Date Created
The date the item was original created (prior to any relationship with the ASU Digital Repositories.)
2024
Agent
- Author (aut): Vaghela, Arpitsinh Rohitkumar
- Thesis advisor (ths): Yang, Yezhou
- Committee member: Lu, Duo
- Committee member: Chakravarthi, Bharatesh
- Committee member: Wei, Hua
- Publisher (pbl): Arizona State University