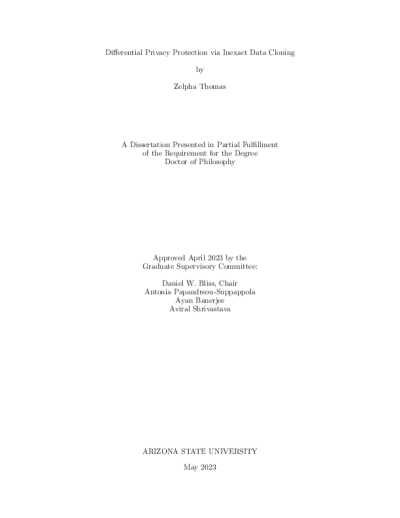
Description
With the advent of new advanced analysis tools and access to related published data, it is getting more difficult for data owners to suppress private information from published data while still providing useful information. This dual problem of providing useful, accurate information and protecting it at the same time has been challenging, especially in healthcare. The data owners lack an automated resource that provides layers of protection on a published dataset with validated statistical values for usability. Differential privacy (DP) has gained a lot of attention in the past few years as a solution to the above-mentioned dual problem. DP is defined as a statistical anonymity model that can protect the data from adversarial observation while still providing intended usage. This dissertation introduces a novel DP protection mechanism called Inexact Data Cloning (IDC), which simultaneously protects and preserves information in published data while conveying source data intent. IDC preserves the privacy of the records by converting the raw data records into clonesets. The clonesets then pass through a classifier that removes potential compromising clonesets, filtering only good inexact cloneset. The mechanism of IDC is dependent on a set of privacy protection metrics called differential privacy protection metrics (DPPM), which represents the overall protection level. IDC uses two novel performance values, differential privacy protection score (DPPS) and clone classifier selection percentage (CCSP), to estimate the privacy level of protected data. In support of using IDC as a viable data security product, a software tool chain prototype, differential privacy protection architecture (DPPA), was developed to utilize the IDC. DPPA used the engineering security mechanism of IDC. DPPA is a hub which facilitates a market for data DP security mechanisms. DPPA works by incorporating standalone IDC mechanisms and provides automation, IDC protected published datasets and statistically verified IDC dataset diagnostic report. DPPA is currently doing functional, and operational benchmark processes that quantifies the DP protection of a given published dataset. The DPPA tool was recently used to test a couple of health datasets. The test results further validate the IDC mechanism as being feasible.
Download count: 6
Details
Title
- Differential Privacy Protection via Inexact Data Cloning
Contributors
- thomas, zelpha (Author)
- Bliss, Daniel W (Thesis advisor)
- Papandreou-Suppappola, Antonia (Committee member)
- Banerjee, Ayan (Committee member)
- Shrivastava, Aviral (Committee member)
- Arizona State University (Publisher)
Date Created
The date the item was original created (prior to any relationship with the ASU Digital Repositories.)
2023
Subjects
Resource Type
Collections this item is in
Note
-
Partial requirement for: Ph.D., Arizona State University, 2023
-
Field of study: Engineering