Computational Challenges in Non-parametric Prediction of Bradycardia in Preterm Infants
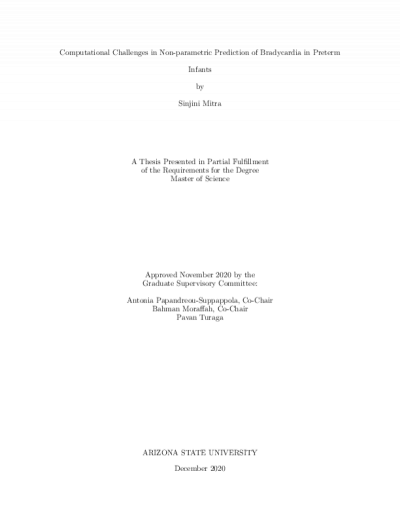
Description
Infants born before 37 weeks of pregnancy are considered to be preterm. Typically, preterm infants have to be strictly monitored since they are highly susceptible to health problems like hypoxemia (low blood oxygen level), apnea, respiratory issues, cardiac problems, neurological problems as well as an increased chance of long-term health issues such as cerebral palsy, asthma and sudden infant death syndrome. One of the leading health complications in preterm infants is bradycardia - which is defined as the slower than expected heart rate, generally beating lower than 60 beats per minute. Bradycardia is often accompanied by low oxygen levels and can cause additional long term health problems in the premature infant.The implementation of a non-parametric method to predict the onset of brady- cardia is presented. This method assumes no prior knowledge of the data and uses kernel density estimation to predict the future onset of bradycardia events. The data is preprocessed, and then analyzed to detect the peaks in the ECG signals, following which different kernels are implemented to estimate the shared underlying distribu- tion of the data. The performance of the algorithm is evaluated using various metrics and the computational challenges and methods to overcome them are also discussed.
It is observed that the performance of the algorithm with regards to the kernels used are consistent with the theoretical performance of the kernel as presented in a previous work. The theoretical approach has also been automated in this work and the various implementation challenges have been addressed.
It is observed that the performance of the algorithm with regards to the kernels used are consistent with the theoretical performance of the kernel as presented in a previous work. The theoretical approach has also been automated in this work and the various implementation challenges have been addressed.
Date Created
The date the item was original created (prior to any relationship with the ASU Digital Repositories.)
2020
Agent
- Author (aut): Mitra, Sinjini
- Thesis advisor (ths): Papandreou-Suppappola, Antonia
- Thesis advisor (ths): Moraffah, Bahman
- Committee member: Turaga, Pavan
- Publisher (pbl): Arizona State University