Integrating Time-Frequency and Machine Learning Methods for Tracking In Radar and Communications Coexisting Systems
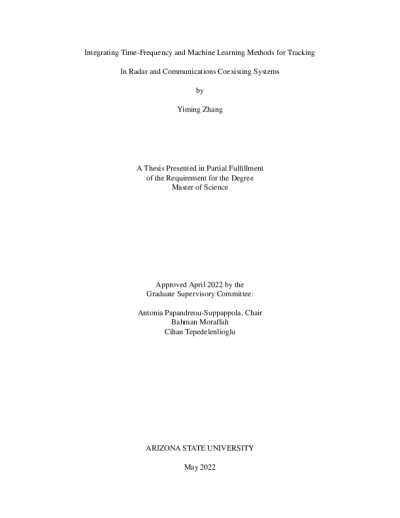
Description
Increased demand on bandwidth has resulted in wireless communications and radar systems sharing spectrum. As signal transmissions from both modalities coexist, methodologies must be designed to reduce the induced interference from each system. This work considers the problem of tracking an object using radar measurements embedded in noise and corrupted from transmissions of multiple communications users. Radar received signals in low noise can be successively processed to estimate object parameters maximum likelihood estimation. For linear frequency-modulated (LFM) signals, such estimates can be efficiently computed by integrating the Wigner distribution along lines in the time-frequency (TF) plane. However, the presence of communications interference highly reduces estimation performance.This thesis proposes a new approach to increase radar estimation performance by integrating a highly-localized TF method with data clustering. The received signal is first decomposed into highly localized Gaussians using the iterative matching pursuit decomposition (MPD). As the MPD is iterative, high noise levels can be reduced by appropriately selecting the algorithm’s stopping criteria. The decomposition also provides feature vectors of reduced dimensionality that can be used for clustering using a Gaussian mixture model (GMM). The proposed estimation method integrates along lines of a modified Wigner distribution of the Gaussian clusters in the TF plane. Using simulations, the object parameter estimation performance of the MPD is shown to highly improve when the MPD is integrated with GMM clustering.
Date Created
The date the item was original created (prior to any relationship with the ASU Digital Repositories.)
2022
Agent
- Author (aut): Zhang, Yiming
- Thesis advisor (ths): Papandreou-Suppappola, Antonia
- Committee member: Moraffah, Bahman
- Committee member: Tepedelenlioğlu, Cihan
- Publisher (pbl): Arizona State University