Collaborative Learning and Optimization for Edge Intelligence
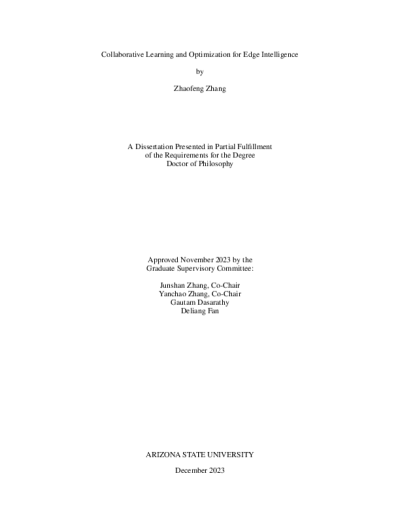
Description
With the proliferation of mobile computing and Internet-of-Things (IoT), billions of mobile and IoT devices are connected to the Internet, generating zillions of Bytes of data at the network edge. Driving by this trend, there is an urgent need to push the artificial intelligence (AI) frontiers to the network edge to unleash the potential of the edge big data fully. This dissertation aims to comprehensively study collaborative learning and optimization algorithms to build a foundation of edge intelligence. Under this common theme, this dissertation is broadly organized into three parts. The first part of this study focuses on model learning with limited data and limited computing capability at the network edge. A global model initialization is first obtained by running federated learning (FL) across many edge devices, based on which a semi-supervised algorithm is devised for an edge device to carry out quick adaptation, aiming to address the insufficiency of labeled data and to learn a personalized model efficiently. In the second part of this study, collaborative learning between the edge and the cloud is studied to achieve real-time edge intelligence. More specifically, a distributionally robust optimization (DRO) approach is proposed to enable the synergy between local data processing and cloud knowledge transfer. Two attractive uncertainty models are investigated corresponding to the cloud knowledge transfer: the distribution uncertainty set based on the cloud data distribution and the prior distribution of the edge model conditioned on the cloud model. Collaborative learning algorithms are developed along this line. The final part focuses on developing an offline model-based safe Inverse Reinforcement Learning (IRL) algorithm for connected Autonomous Vehicles (AVs). A reward penalty is introduced to penalize unsafe states, and a risk-measure-based approach is proposed to mitigate the model uncertainty introduced by offline training. The experimental results demonstrate the improvement of the proposed algorithm over the existing baselines in terms of cumulative rewards.
Date Created
The date the item was original created (prior to any relationship with the ASU Digital Repositories.)
2023
Agent
- Author (aut): Zhang, Zhaofeng
- Thesis advisor (ths): Zhang, Junshan
- Thesis advisor (ths): Zhang, Yanchao
- Committee member: Dasarathy, Gautam
- Committee member: Fan, Deliang
- Publisher (pbl): Arizona State University