Microstructural Control in Fabricating Multifunctional Carbon Fibers
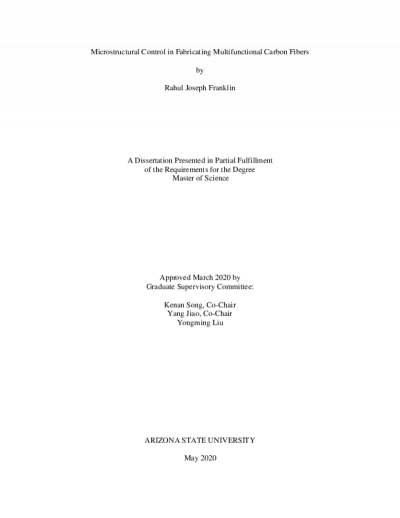
Description
Precursors of carbon fibers include rayon, pitch, and polyacrylonitrile fibers that can be heat-treated for high-strength or high-modulus carbon fibers. Among them, polyacrylonitrile has been used most frequently due to its low viscosity for easy processing and excellent performance for high-end applications. To further explore polyacrylonitrile-based fibers for better precursors, in this study, carbon nanofillers were introduced in the polymer matrix to examine their reinforcement effects and influences on carbon fiber performance. Two-dimensional graphene nanoplatelets were mainly used for the polymer reinforcement and one-dimensional carbon nanotubes were also incorporated in polyacrylonitrile as a comparison. Dry-jet wet spinning was used to fabricate the composite fibers. Hot-stage drawing and heat-treatment were used to evolve the physical microstructures and molecular morphologies of precursor and carbon fibers. As compared to traditionally used random dispersions, selective placement of nanofillers was effective in improving composite fiber properties and enhancing mechanical and functional behaviors of carbon fibers. The particular position of reinforcement fillers with polymer layers was enabled by the in-house developed spinneret used for fiber spinning. The preferential alignment of graphitic planes contributed to the enhanced mechanical and functional behaviors than those of dispersed nanoparticles in polyacrylonitrile composites. The high in-plane modulus of graphene and the induction to polyacrylonitrile molecular carbonization/graphitization were the motivation for selectively placing graphene nanoplatelets between polyacrylonitrile layers. Mechanical tests, scanning electron microscopy, thermal, and electrical properties were characterized. Applications such as volatile organic compound sensing and pressure sensing were demonstrated.
Date Created
The date the item was original created (prior to any relationship with the ASU Digital Repositories.)
2020
Agent
- Author (aut): Franklin, Rahul Joseph
- Thesis advisor (ths): Song, Kenan
- Thesis advisor (ths): Jiao, Yang
- Committee member: Liu, Yongming
- Publisher (pbl): Arizona State University