A Framework for the Design and Optimization of Composites with Electromagnetic Wave Scattering Properties Utilizing Two-Point Spatial Correlation Functions
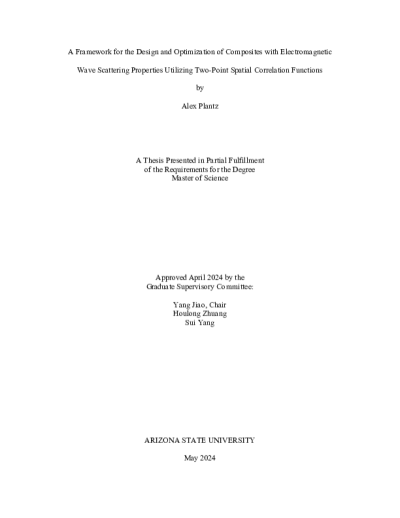
Description
In the age of 5th and upcoming 6th generation fighter aircraft one key proponent of these impressive machines is the inclusion of stealth. This inclusion is demonstrated by thoughtful design pertaining to the shape of the aircraft and rigorous material selection. Both criteria aim to minimize the radar cross section of these aircraft over a wide bandwidth of frequencies corresponding to an ever-evolving field of radar technology. Stealth is both an offensive and defensive capability meaning that service men and women depend on this feature to carry out their missions, and to return home safely. The goal of this paper is to introduce a novel method to designing disordered two-phase composites with desired electromagnetic properties. This task is accomplished by employing the spatial point correlation function, specifically at the two-point level. Effective at describing the dispersion of phases within a two-phase system, the two-point correlation function serves as a statistical function that becomes a realizable target for heterogeneous composites. Simulated annealing is exercised to reconstruct two-phase composite microstructures that initially do not match their target function, followed by two separate experiments aimed at studying the impact of the provided inputs on its outcome. Once conditions for reconstructing highly accurate microstructures are identified, modifications are made to the target function to extract and compare dielectric constants associated with each microstructure. Both the real and imaginary components, which respectively affect wave propagation and attenuation, of the dielectric constants are plotted to illustrate their behavior with increasing wavenumber. Conclusions suggest that favorable values of the complex dielectric constant can be reverse-engineered via careful consideration of the two-point correlation function. Subsequently, corresponding microstructures of the composite can be simulated and then produced through 3-D printing for testing and practical applications.
Date Created
The date the item was original created (prior to any relationship with the ASU Digital Repositories.)
2024
Agent
- Author (aut): Plantz, Alex Chadewick
- Thesis advisor (ths): Jiao, Yang
- Committee member: Zhuang, Houlong
- Committee member: Yang, Sui
- Publisher (pbl): Arizona State University