Automatic Program Optimization by Semantic Relaxation for Parallel Processing Accelerators
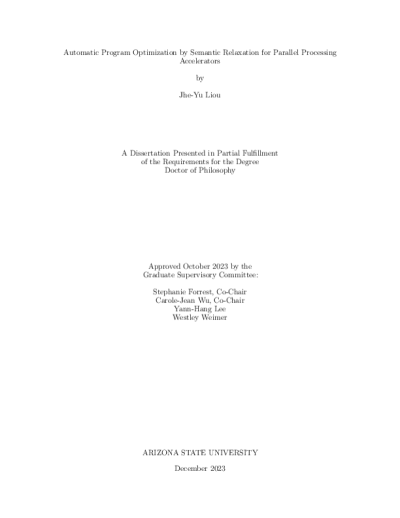
Description
Graphic Processing Units (GPUs) have become a key enabler of the big-data revolution, functioning as defacto co-processors to accelerate large-scale computation. As the GPU programming stack and tool support have matured, the technology has alsobecome accessible to programmers. However, optimizing programs to run efficiently on GPUs requires developers to have both detailed understanding of the application logic and significant knowledge of parallel programming and GPU architectures.
This dissertation proposes GEVO, a tool for automatically tuning the performance of GPU kernels in the LLVM representation to meet desired criteria. GEVO uses population-based search to find edits to programs compiled to LLVM-IR which improves performance on desired criteria and retains required functionality. The evaluation of GEVO on the Rodinia benchmark suite demonstrates many runtime optimization techniques. One of the key insights is that semantic relaxation enables GEVO to
discover these optimizations that are usually prohibited by the compiler. GEVO also explores many other optimizations, including architecture- and application-specific. A follow-up evaluation of three bioinformatics applications at their different stages of development suggests that GEVO can optimize programs as well as human experts, sometimes even into a code base that is beyond a programmer’s reach. Furthermore, to unshackle the constraint of GEVO in optimizing neural network (NN) models,
GEVO-ML is proposed by extending the representation capability of GEVO, where NN models and the training/prediction process are uniformly represented in a single intermediate language. An evaluation of GEVO-ML shows that GEVO-ML can
optimize network models similar to how human developers improve model design, for example, by changing learning rates or pruning non-essential parameters. These results showcase the potential of automated program optimization tools to both reduce
the optimization burden for researchers and provide new insights for GPU experts.
Date Created
The date the item was original created (prior to any relationship with the ASU Digital Repositories.)
2023
Agent
- Author (aut): Liou, Jhe-Yu
- Thesis advisor (ths): Forrest, Stephanie
- Thesis advisor (ths): Wu, Carole-Jean
- Committee member: Lee, Yann-Hang
- Committee member: Weimer, Westley
- Publisher (pbl): Arizona State University