Roundabout Dilemma Zone Detection with Trajectory Forecasting
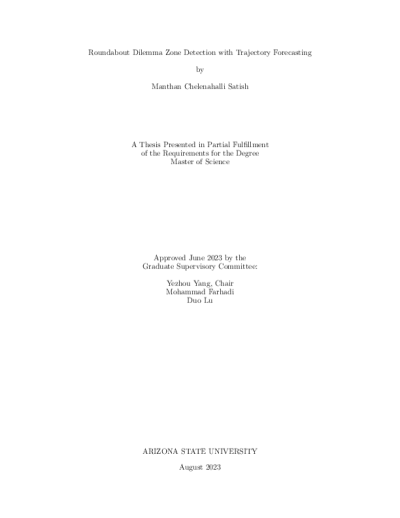
Description
In recent years, there has been a growing emphasis on developing automated systems to enhance traffic safety, particularly in the detection of dilemma zones (DZ) at intersections. This study focuses on the automated detection of DZs at roundabouts using trajectory forecasting, presenting an advanced system with perception capabilities. The system utilizes a modular, graph-structured recurrent model that predicts the trajectories of various agents, accounting for agent dynamics and incorporating heterogeneous data such as semantic maps. This enables the system to facilitate traffic management decision-making and improve overall intersection safety. To assess the system's performance, a real-world dataset of traffic roundabout intersections was employed. The experimental results demonstrate that our Superpowered Trajectron++ system exhibits high accuracy in detecting DZ events, with a false positive rate of approximately 10%. Furthermore, the system has the remarkable ability to anticipate and identify dilemma events before they occur, enabling it to provide timely instructions to vehicles. These instructions serve as guidance, determining whether vehicles should come to a halt or continue moving through the intersection, thereby enhancing safety and minimizing potential conflicts. In summary, the development of automated systems for detecting DZs represents an important advancement in traffic safety. The Superpowered Trajectron++ system, with its trajectory forecasting capabilities and incorporation of diverse data sources, showcases improved accuracy in identifying DZ events and can effectively guide vehicles in making informed decisions at roundabout intersections.
Date Created
The date the item was original created (prior to any relationship with the ASU Digital Repositories.)
2023
Agent
- Author (aut): Chelenahalli Satish, Manthan
- Thesis advisor (ths): Yang, Yezhou
- Committee member: Lu, Duo
- Committee member: Farhadi, Mohammad
- Publisher (pbl): Arizona State University