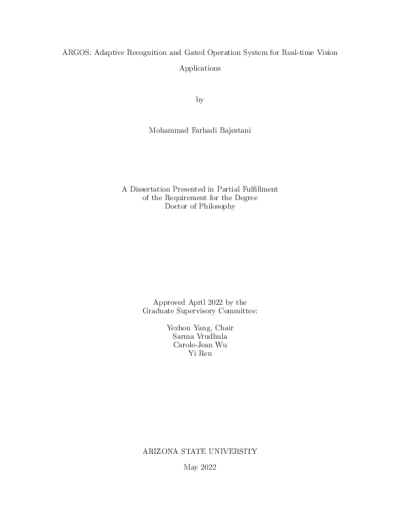
Description
Deep neural network-based methods have been proved to achieve outstanding performance on object detection and classification tasks. Deep neural networks follow the ``deeper model with deeper confidence'' belief to gain a higher recognition accuracy. However, reducing these networks' computational costs remains a challenge, which impedes their deployment on embedded devices. For instance, the intersection management of Connected Autonomous Vehicles (CAVs) requires running computationally intensive object recognition algorithms on low-power traffic cameras. This dissertation aims to study the effect of a dynamic hardware and software approach to address this issue. Characteristics of real-world applications can facilitate this dynamic adjustment and reduce the computation. Specifically, this dissertation starts with a dynamic hardware approach that adjusts itself based on the toughness of input and extracts deeper features if needed. Next, an adaptive learning mechanism has been studied that use extracted feature from previous inputs to improve system performance. Finally, a system (ARGOS) was proposed and evaluated that can be run on embedded systems while maintaining the desired accuracy. This system adopts shallow features at inference time, but it can switch to deep features if the system desires a higher accuracy. To improve the performance, ARGOS distills the temporal knowledge from deep features to the shallow system. Moreover, ARGOS reduces the computation furthermore by focusing on regions of interest. The response time and mean average precision are adopted for the performance evaluation to evaluate the proposed ARGOS system.
Details
Title
- ARGOS: Adaptive Recognition and Gated Operation System for Real-time Vision Applications
Contributors
- Farhadi, Mohammad (Author)
- Yang, Yezhou (Thesis advisor)
- Vrudhula, Sarma (Committee member)
- Wu, Carole-Jean (Committee member)
- Ren, Yi (Committee member)
- Arizona State University (Publisher)
Date Created
The date the item was original created (prior to any relationship with the ASU Digital Repositories.)
2022
Subjects
Resource Type
Collections this item is in
Note
- Partial requirement for: Ph.D., Arizona State University, 2022
- Field of study: Computer Engineering