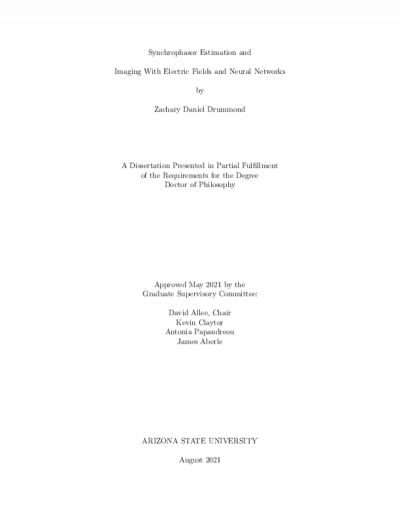
Description
This research presents advances in time-synchronized phasor (i.e.,synchrophasor) estimation and imaging with very-low-frequency electric fields.
Phasor measurement units measure and track dynamic systems, often power
systems, using synchrophasor estimation algorithms. Two improvements to
subspace-based synchrophasor estimation algorithms are shown. The first
improvement is a dynamic thresholding method for accurately determining the
signal subspace when using the estimation of signal parameters via rotational
invariance techniques (ESPRIT) algorithm. This improvement facilitates
accurate ESPRIT-based frequency estimates of both the nominal system frequency
and the frequencies of interfering signals such as harmonics or out-of-band
interference signals. Proper frequency estimation of all signals present in
measurement data allows for accurate least squares estimates of synchrophasors
for the nominal system frequency. By including the effects of clutter signals
in the synchrophasor estimate, interference from clutter signals can be
excluded. The result is near-flat estimation error during nominal system
frequency changes, the presence of harmonic distortion, and out-of-band
interference. The second improvement reduces the computational burden of the
ESPRIT frequency estimation step by showing that an optimized Eigenvalue
decomposition of the measurement data can be used instead of a singular value
decomposition. This research also explores a deep-learning-based inversion
method for imaging objects with a uniform electric field and a 2D planar D-dot
array. Using electric fields as an illumination source has seen multiple
applications ranging from medical imaging to mineral deposit detection. It is
shown that a planar D-dot array and deep neural network can reconstruct the
electrical properties of randomized objects. A 16000-sample dataset of objects
comprised of a three-by-three grid of randomized dielectric constants was
generated to train a deep neural network for predicting these dielectric
constants from measured field distortions. Increasingly complex imaging
environments are simulated, ranging from objects in free space to objects
placed in a physical cage designed to produce uniform electric fields.
Finally, this research relaxes the uniform electric field constraint, showing
that the volume of an opaque container can be imaged with a copper tube antenna
and a 1x4 array of D-dot sensors. Real world experimental results
show that it is possible to image buckets of water (targets) within a plastic
shed These experiments explore the detectability of targets as a function of
target placement within the shed.
Details
Title
- Synchrophasor Estimation and Imaging With Electric Fields and Neural Networks
Contributors
- Drummond, Zachary (Author)
- Allee, David R (Thesis advisor)
- Claytor, Kevin E (Committee member)
- Papandreou-Suppappola, Antonia (Committee member)
- Aberle, James (Committee member)
- Arizona State University (Publisher)
Date Created
The date the item was original created (prior to any relationship with the ASU Digital Repositories.)
2021
Subjects
Resource Type
Collections this item is in
Note
-
Partial requirement for: Ph.D., Arizona State University, 2021
-
Field of study: Electrical Engineering