Control of Unmanned Aerial Vehicles for Mission Critical Tasks
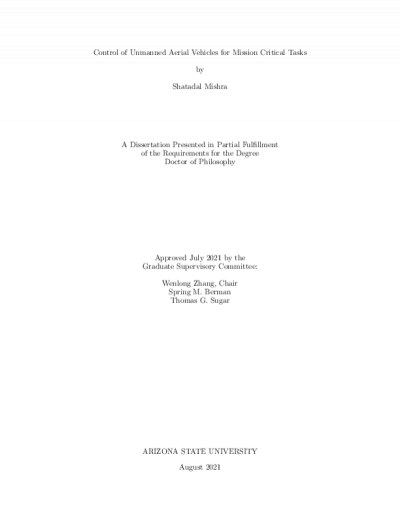
Description
Unmanned aerial vehicles (UAVs) have reshaped the world of aviation. With the emergence of different types of UAVs, a multitude of mission critical applications, e.g., aerial photography, package delivery, grasping and manipulation, aerial reconnaissance and surveillance have been accomplished successfully. All of the aforementioned applications require the UAVs to be robust to external disturbances and safe while flying in cluttered environments and these factors are of paramount importance for task completion. In the first phase, this dissertation starts by presenting the synthesis and experimental validation of real-time low-level estimation and robust attitude and position controllers for multirotors. For the task of reliable position estimation, a hybrid low-pass de-trending filter is proposed for attenuating noise and drift in the velocity and position estimates respectively. Subsequently, a disturbance observer (DOB) approach with online Q-filter tuning is proposed for disturbance rejection and precise position control. Finally, a non-linear disturbance observer (NDOB) approach, along with a parameter optimization framework, is proposed for robust attitude control of multirotors. Multiple simulation and experimental flight tests are performed to demonstrate the efficacy of the proposed algorithms. Aerial grasping and collection is a type of mission-critical task which requires vision based sensing and robust control algorithms for successful task completion. In the second phase, this dissertation initially explores different object grasping approaches utilizing soft and rigid graspers. Additionally, vision based control paradigms are developed for object grasping and collection applications, specifically from water surfaces. Autonomous object collection from water surfaces presents a multitude of challenges: i) object drift due to propeller outwash, ii) reflection and glare from water surfaces makes object detection extremely challenging and iii) lack of reliable height sensors above water surface (for autonomous landing on water). Finally, a first of its kind aerial manipulation system, with an integrated net system and a robust vision based control structure, is proposed for floating object collection from water surfaces. Objects of different shapes and sizes are collected, through multiple experimental flight tests, with a success rate of 91.6%. To the best of the author's knowledge, this is the first work demonstrating autonomous object collection from water surfaces.
Date Created
The date the item was original created (prior to any relationship with the ASU Digital Repositories.)
2021
Agent
- Author (aut): Mishra, Shatadal
- Thesis advisor (ths): Zhang, Wenlong
- Committee member: Berman, Spring M
- Committee member: Sugar, Thomas G
- Publisher (pbl): Arizona State University