Harnessing Structure in Discrete and Non-convex optimization with applications in online learning, multi-agent systems, and phase retrieval
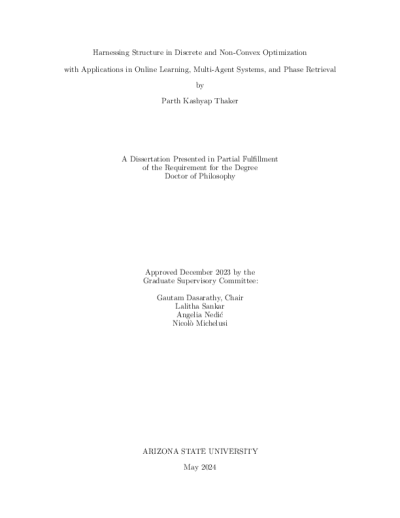
Description
This thesis examines the critical relationship between data, complex models, and other methods to measure and analyze them. As models grow larger and more intricate, they require more data, making it vital to use that data effectively. The document starts with a deep dive into nonconvex functions, a fundamental element of modern complex systems, identifying key conditions that ensure these systems can be analyzed efficiently—a crucial consideration in an era of vast amounts of variables. Loss functions, traditionally seen as mere optimization tools, are analyzed and recast as measures of how accurately a model reflects reality. This redefined perspective permits the refinement of data-sourcing strategies for a better data economy. The aim of the investigation is the model itself, which is used to understand and harness the underlying patterns of complex systems. By incorporating structure both implicitly (through periodic patterns) and explicitly (using graphs), the model's ability to make sense of the data is enhanced. Moreover, online learning principles are applied to a crucial practical scenario: robotic resource monitoring. The results established in this thesis, backed by simulations and theoretical proofs, highlight the advantages of online learning methods over traditional ones commonly used in robotics. In sum, this thesis presents an integrated approach to measuring complex systems, providing new insights and methods that push forward the capabilities of machine learning.
Date Created
The date the item was original created (prior to any relationship with the ASU Digital Repositories.)
2024
Agent
- Author (aut): Thaker, Parth Kashyap
- Thesis advisor (ths): Dasarathy, Gautam
- Committee member: Sankar, Lalitha
- Committee member: Nedich, Angelia
- Committee member: Michelusi, Nicolò
- Publisher (pbl): Arizona State University