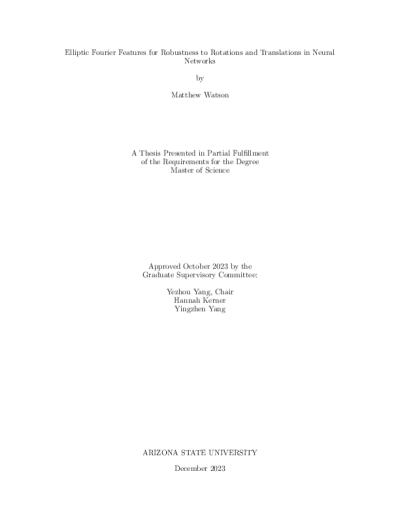
Description
In image classification tasks, images are often corrupted by spatial transformationslike translations and rotations. In this work, I utilize an existing method that uses
the Fourier series expansion to generate a rotation and translation invariant representation of closed contours found in sketches, aiming to attenuate the effects of distribution shift caused by the aforementioned transformations. I use this technique to
transform input images into one of two different invariant representations, a Fourier
series representation and a corrected raster image representation, prior to passing
them to a neural network for classification. The architectures used include convolutional neutral networks (CNNs), multi-layer perceptrons (MLPs), and graph neural
networks (GNNs). I compare the performance of this method to using data augmentation during training, the standard approach for addressing distribution shift, to see
which strategy yields the best performance when evaluated against a test set with
rotations and translations applied. I include experiments where the augmentations
applied during training both do and do not accurately reflect the transformations encountered at test time. Additionally, I investigate the robustness of both approaches
to high-frequency noise. In each experiment, I also compare training efficiency across
models. I conduct experiments on three data sets, the MNIST handwritten digit
dataset, a custom dataset (QD-3) consisting of three classes of geometric figures from
the Quick, Draw! hand-drawn sketch dataset, and another custom dataset (QD-345)
featuring sketches from all 345 classes found in Quick, Draw!. On the smaller problem space of MNIST and QD-3, the networks utilizing the Fourier-based technique to
attenuate distribution shift perform competitively with the standard data augmentation strategy. On the more complex problem space of QD-345, the networks using the
Fourier technique do not achieve the same test performance as correctly-applied data
augmentation. However, they still outperform instances where train-time augmentations mis-predict test-time transformations, and outperform a naive baseline model
where no strategy is used to attenuate distribution shift. Overall, this work provides
evidence that strategies which attempt to directly mitigate distribution shift, rather
than simply increasing the diversity of the training data, can be successful when
certain conditions hold.
Details
Title
- Elliptic Fourier Features for Robustness to Rotations and Translations in Neural Networks
Contributors
- Watson, Matthew (Author)
- Yang, Yezhou YY (Thesis advisor)
- Kerner, Hannah HK (Committee member)
- Yang, Yingzhen YY (Committee member)
- Arizona State University (Publisher)
Date Created
The date the item was original created (prior to any relationship with the ASU Digital Repositories.)
2023
Subjects
Resource Type
Collections this item is in
Note
- Partial requirement for: M.S., Arizona State University, 2023
- Field of study: Computer Science