Wireless Charging Technologies
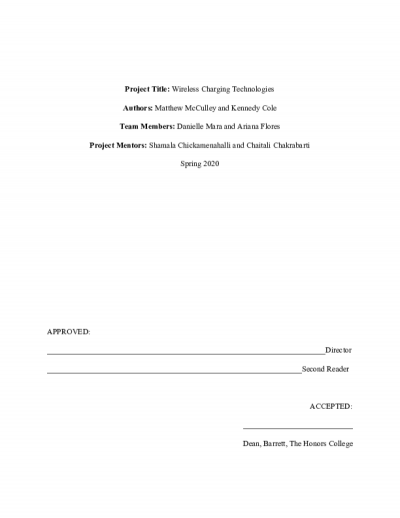
Description
In the world we live in today, nothing is impossible. Due to the advancements of technology, humans around the globe are able to hold computers that fit within the size of their pocket. These computers can do marvelous things, however run off batteries. These batteries need to be charged and up until a little while ago there was only one option available: wired chargers; however, because of the advancement of technology society has created a way to transfer power via magnetic fields. Now this concept has been around for a long time since the days of Nikola Tesla but just recently society has been able to apply his discoveries to charging these computers in our pockets. Unfortunately, the current models of these chargers come with a drawback as they are less efficient than wired chargers. However, this is the question our group has set out to answer. Is there any way possible to improve the efficiency of these wireless chargers so they are equal or even more efficient than wired chargers. This paper explores how to improve the efficiency in wireless chargers. Through research, simulations and testing the group has discovered areas that efficiency can be improved as well as makes recommendations to change the current wireless chargers on the market today. This paper also explores future applications of wireless chargers that can not only make life much easier but could also save lives in some cases. These applications can have many effects on hospitality, the medical field, as well as the supply chain and logistics of America.
Date Created
The date the item was original created (prior to any relationship with the ASU Digital Repositories.)
2020-05
Agent
- Co-author: McCulley, Matthew Alan
- Co-author: Cole, Kennedy
- Thesis director: Chickamenahalli, Shamala
- Committee member: Chakrabarti, Chaitali
- Contributor (ctb): Electrical Engineering Program
- Contributor (ctb): Barrett, The Honors College