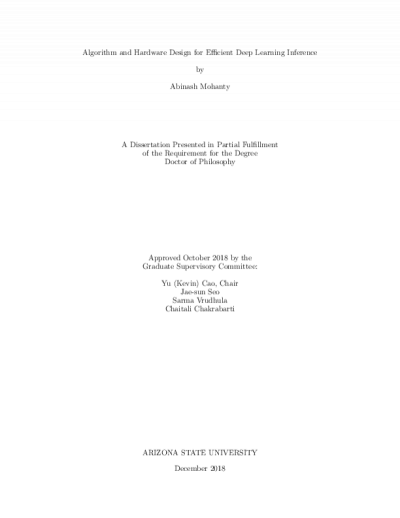
Description
Deep learning (DL) has proved itself be one of the most important developements till date with far reaching impacts in numerous fields like robotics, computer vision, surveillance, speech processing, machine translation, finance, etc. They are now widely used for countless applications because of their ability to generalize real world data, robustness to noise in previously unseen data and high inference accuracy. With the ability to learn useful features from raw sensor data, deep learning algorithms have out-performed tradinal AI algorithms and pushed the boundaries of what can be achieved with AI. In this work, we demonstrate the power of deep learning by developing a neural network to automatically detect cough instances from audio recorded in un-constrained environments. For this, 24 hours long recordings from 9 dierent patients is collected and carefully labeled by medical personel. A pre-processing algorithm is proposed to convert event based cough dataset to a more informative dataset with start and end of coughs and also introduce data augmentation for regularizing the training procedure. The proposed neural network achieves 92.3% leave-one-out accuracy on data captured in real world.
Deep neural networks are composed of multiple layers that are compute/memory intensive. This makes it difficult to execute these algorithms real-time with low power consumption using existing general purpose computers. In this work, we propose hardware accelerators for a traditional AI algorithm based on random forest trees and two representative deep convolutional neural networks (AlexNet and VGG). With the proposed acceleration techniques, ~ 30x performance improvement was achieved compared to CPU for random forest trees. For deep CNNS, we demonstrate that much higher performance can be achieved with architecture space exploration using any optimization algorithms with system level performance and area models for hardware primitives as inputs and goal of minimizing latency with given resource constraints. With this method, ~30GOPs performance was achieved for Stratix V FPGA boards.
Hardware acceleration of DL algorithms alone is not always the most ecient way and sucient to achieve desired performance. There is a huge headroom available for performance improvement provided the algorithms are designed keeping in mind the hardware limitations and bottlenecks. This work achieves hardware-software co-optimization for Non-Maximal Suppression (NMS) algorithm. Using the proposed algorithmic changes and hardware architecture
With CMOS scaling coming to an end and increasing memory bandwidth bottlenecks, CMOS based system might not scale enough to accommodate requirements of more complicated and deeper neural networks in future. In this work, we explore RRAM crossbars and arrays as compact, high performing and energy efficient alternative to CMOS accelerators for deep learning training and inference. We propose and implement RRAM periphery read and write circuits and achieved ~3000x performance improvement in online dictionary learning compared to CPU.
This work also examines the realistic RRAM devices and their non-idealities. We do an in-depth study of the effects of RRAM non-idealities on inference accuracy when a pretrained model is mapped to RRAM based accelerators. To mitigate this issue, we propose Random Sparse Adaptation (RSA), a novel scheme aimed at tuning the model to take care of the faults of the RRAM array on which it is mapped. Our proposed method can achieve inference accuracy much higher than what traditional Read-Verify-Write (R-V-W) method could achieve. RSA can also recover lost inference accuracy 100x ~ 1000x faster compared to R-V-W. Using 32-bit high precision RSA cells, we achieved ~10% higher accuracy using fautly RRAM arrays compared to what can be achieved by mapping a deep network to an 32 level RRAM array with no variations.
Deep neural networks are composed of multiple layers that are compute/memory intensive. This makes it difficult to execute these algorithms real-time with low power consumption using existing general purpose computers. In this work, we propose hardware accelerators for a traditional AI algorithm based on random forest trees and two representative deep convolutional neural networks (AlexNet and VGG). With the proposed acceleration techniques, ~ 30x performance improvement was achieved compared to CPU for random forest trees. For deep CNNS, we demonstrate that much higher performance can be achieved with architecture space exploration using any optimization algorithms with system level performance and area models for hardware primitives as inputs and goal of minimizing latency with given resource constraints. With this method, ~30GOPs performance was achieved for Stratix V FPGA boards.
Hardware acceleration of DL algorithms alone is not always the most ecient way and sucient to achieve desired performance. There is a huge headroom available for performance improvement provided the algorithms are designed keeping in mind the hardware limitations and bottlenecks. This work achieves hardware-software co-optimization for Non-Maximal Suppression (NMS) algorithm. Using the proposed algorithmic changes and hardware architecture
With CMOS scaling coming to an end and increasing memory bandwidth bottlenecks, CMOS based system might not scale enough to accommodate requirements of more complicated and deeper neural networks in future. In this work, we explore RRAM crossbars and arrays as compact, high performing and energy efficient alternative to CMOS accelerators for deep learning training and inference. We propose and implement RRAM periphery read and write circuits and achieved ~3000x performance improvement in online dictionary learning compared to CPU.
This work also examines the realistic RRAM devices and their non-idealities. We do an in-depth study of the effects of RRAM non-idealities on inference accuracy when a pretrained model is mapped to RRAM based accelerators. To mitigate this issue, we propose Random Sparse Adaptation (RSA), a novel scheme aimed at tuning the model to take care of the faults of the RRAM array on which it is mapped. Our proposed method can achieve inference accuracy much higher than what traditional Read-Verify-Write (R-V-W) method could achieve. RSA can also recover lost inference accuracy 100x ~ 1000x faster compared to R-V-W. Using 32-bit high precision RSA cells, we achieved ~10% higher accuracy using fautly RRAM arrays compared to what can be achieved by mapping a deep network to an 32 level RRAM array with no variations.
Details
Title
- Algorithm and Hardware Design for Efficient Deep Learning Inference
Contributors
- Mohanty, Abinash (Author)
- Cao, Yu (Thesis advisor)
- Seo, Jae-Sun (Committee member)
- Vrudhula, Sarma (Committee member)
- Chakrabarti, Chaitali (Committee member)
- Arizona State University (Publisher)
Date Created
The date the item was original created (prior to any relationship with the ASU Digital Repositories.)
2018
Subjects
Resource Type
Collections this item is in
Note
- Doctoral Dissertation Electrical Engineering 2018