Low Cost 3D Flow Estimation in Medical Ultrasound
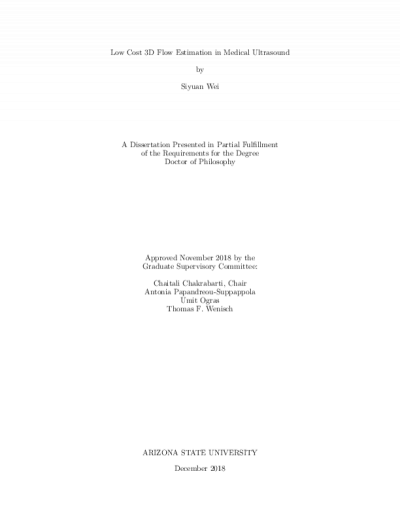
Description
Medical ultrasound imaging is widely used today because of it being non-invasive and cost-effective. Flow estimation helps in accurate diagnosis of vascular diseases and adds an important dimension to medical ultrasound imaging. Traditionally flow estimation is done using Doppler-based methods which only estimate velocity in the beam direction. Thus when blood vessels are close to being orthogonal to the beam direction, there are large errors in the estimation results. In this dissertation, a low cost blood flow estimation method that does not have the angle dependency of Doppler-based methods, is presented.
First, a velocity estimator based on speckle tracking and synthetic lateral phase is proposed for clutter-free blood flow.
Speckle tracking is based on kernel matching and does not have any angle dependency. While velocity estimation in axial dimension is accurate, lateral velocity estimation is challenging due to reduced resolution and lack of phase information. This work presents a two tiered method which estimates the pixel level movement using sum-of-absolute difference, and then estimates the sub-pixel level using synthetic phase information in the lateral dimension. Such a method achieves highly accurate velocity estimation with reduced complexity compared to a cross correlation based method. The average bias of the proposed estimation method is less than 2% for plug flow and less than 7% for parabolic flow.
Blood is always accompanied by clutter which originates from vessel wall and surrounding tissues. As magnitude of the blood signal is usually 40-60 dB lower than magnitude of the clutter signal, clutter filtering is necessary before blood flow estimation. Clutter filters utilize the high magnitude and low frequency features of clutter signal to effectively remove them from the compound (blood + clutter) signal. Instead of low complexity FIR filter or high complexity SVD-based filters, here a power/subspace iteration based method is proposed for clutter filtering. Excellent clutter filtering performance is achieved for both slow and fast moving clutters with lower complexity compared to SVD-based filters. For instance, use of the proposed method results in the bias being less than 8% and standard deviation being less than 12% for fast moving clutter when the beam-to-flow-angle is $90^o$.
Third, a flow rate estimation method based on kernel power weighting is proposed. As the velocity estimator is a kernel-based method, the estimation accuracy degrades near the vessel boundary. In order to account for kernels that are not fully inside the vessel, fractional weights are given to these kernels based on their signal power. The proposed method achieves excellent flow rate estimation results with less than 8% bias for both slow and fast moving clutters.
The performance of the velocity estimator is also evaluated for challenging models. A 2D version of our two-tiered method is able to accurately estimate velocity vectors in a spinning disk as well as in a carotid bifurcation model, both of which are part of the synthetic aperture vector flow imaging (SA-VFI) challenge of 2018. In fact, the proposed method ranked 3rd in the challenge for testing dataset with carotid bifurcation. The flow estimation method is also evaluated for blood flow in vessels with stenosis. Simulation results show that the proposed method is able to estimate the flow rate with less than 9% bias.
First, a velocity estimator based on speckle tracking and synthetic lateral phase is proposed for clutter-free blood flow.
Speckle tracking is based on kernel matching and does not have any angle dependency. While velocity estimation in axial dimension is accurate, lateral velocity estimation is challenging due to reduced resolution and lack of phase information. This work presents a two tiered method which estimates the pixel level movement using sum-of-absolute difference, and then estimates the sub-pixel level using synthetic phase information in the lateral dimension. Such a method achieves highly accurate velocity estimation with reduced complexity compared to a cross correlation based method. The average bias of the proposed estimation method is less than 2% for plug flow and less than 7% for parabolic flow.
Blood is always accompanied by clutter which originates from vessel wall and surrounding tissues. As magnitude of the blood signal is usually 40-60 dB lower than magnitude of the clutter signal, clutter filtering is necessary before blood flow estimation. Clutter filters utilize the high magnitude and low frequency features of clutter signal to effectively remove them from the compound (blood + clutter) signal. Instead of low complexity FIR filter or high complexity SVD-based filters, here a power/subspace iteration based method is proposed for clutter filtering. Excellent clutter filtering performance is achieved for both slow and fast moving clutters with lower complexity compared to SVD-based filters. For instance, use of the proposed method results in the bias being less than 8% and standard deviation being less than 12% for fast moving clutter when the beam-to-flow-angle is $90^o$.
Third, a flow rate estimation method based on kernel power weighting is proposed. As the velocity estimator is a kernel-based method, the estimation accuracy degrades near the vessel boundary. In order to account for kernels that are not fully inside the vessel, fractional weights are given to these kernels based on their signal power. The proposed method achieves excellent flow rate estimation results with less than 8% bias for both slow and fast moving clutters.
The performance of the velocity estimator is also evaluated for challenging models. A 2D version of our two-tiered method is able to accurately estimate velocity vectors in a spinning disk as well as in a carotid bifurcation model, both of which are part of the synthetic aperture vector flow imaging (SA-VFI) challenge of 2018. In fact, the proposed method ranked 3rd in the challenge for testing dataset with carotid bifurcation. The flow estimation method is also evaluated for blood flow in vessels with stenosis. Simulation results show that the proposed method is able to estimate the flow rate with less than 9% bias.
Date Created
The date the item was original created (prior to any relationship with the ASU Digital Repositories.)
2018
Agent
- Author (aut): Wei, Siyuan
- Thesis advisor (ths): Chakrabarti, Chaitali
- Committee member: Papandreou-Suppappola, Antonia
- Committee member: Ogras, Umit Y.
- Committee member: Wenisch, Thomas F.
- Publisher (pbl): Arizona State University