Global Optimization Using Piecewise Linear Approximation
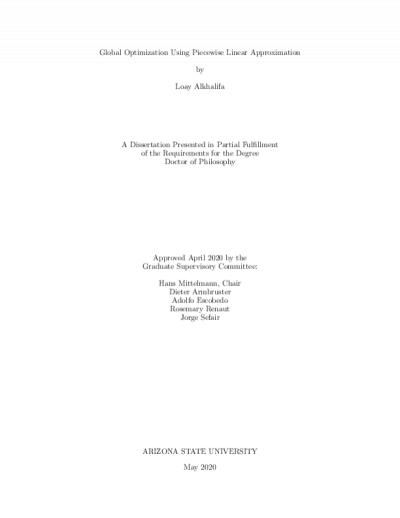
Description
Global optimization (programming) has been attracting the attention of researchers for almost a century. Since linear programming (LP) and mixed integer linear programming (MILP) had been well studied in early stages, MILP methods and software tools had improved in their efficiency in the past few years. They are now fast and robust even for problems with millions of variables. Therefore, it is desirable to use MILP software to solve mixed integer nonlinear programming (MINLP) problems. For an MINLP problem to be solved by an MILP solver, its nonlinear functions must be transformed to linear ones. The most common method to do the transformation is the piecewise linear approximation (PLA). This dissertation will summarize the types of optimization and the most important tools and methods, and will discuss in depth the PLA tool. PLA will be done using nonuniform partitioning of the domain of the variables involved in the function that will be approximated. Also partial PLA models that approximate only parts of a complicated optimization problem will be introduced. Computational experiments will be done and the results will show that nonuniform partitioning and partial PLA can be beneficial.
Date Created
The date the item was original created (prior to any relationship with the ASU Digital Repositories.)
2020
Agent
- Author (aut): Alkhalifa, Loay
- Thesis advisor (ths): Mittelmann, Hans
- Committee member: Armbruster, Hans
- Committee member: Escobedo, Adolfo
- Committee member: Renaut, Rosemary
- Committee member: Sefair, Jorge
- Publisher (pbl): Arizona State University