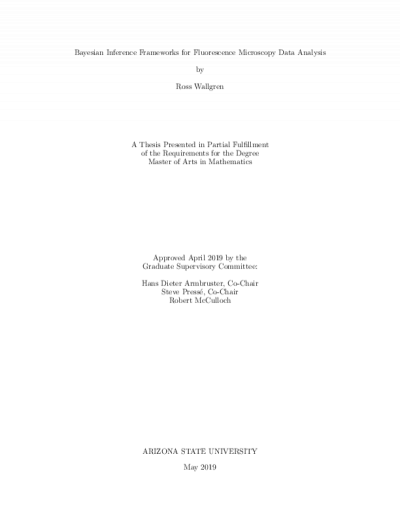
Description
In this work, I present a Bayesian inference computational framework for the analysis of widefield microscopy data that addresses three challenges: (1) counting and localizing stationary fluorescent molecules; (2) inferring a spatially-dependent effective fluorescence profile that describes the spatially-varying rate at which fluorescent molecules emit subsequently-detected photons (due to different illumination intensities or different local environments); and (3) inferring the camera gain. My general theoretical framework utilizes the Bayesian nonparametric Gaussian and beta-Bernoulli processes with a Markov chain Monte Carlo sampling scheme, which I further specify and implement for Total Internal Reflection Fluorescence (TIRF) microscopy data, benchmarking the method on synthetic data. These three frameworks are self-contained, and can be used concurrently so that the fluorescence profile and emitter locations are both considered unknown and, under some conditions, learned simultaneously. The framework I present is flexible and may be adapted to accommodate the inference of other parameters, such as emission photophysical kinetics and the trajectories of moving molecules. My TIRF-specific implementation may find use in the study of structures on cell membranes, or in studying local sample properties that affect fluorescent molecule photon emission rates.
Details
Title
- Bayesian Inference Frameworks for Fluorescence Microscopy Data Analysis
Contributors
- Wallgren, Ross (Author)
- Presse, Steve (Thesis advisor)
- Armbruster, Hans (Thesis advisor)
- McCulloch, Robert (Committee member)
- Arizona State University (Publisher)
Date Created
The date the item was original created (prior to any relationship with the ASU Digital Repositories.)
2019
Subjects
- Mathematics
- Statistics
- Biophysics
- Bayesian
- beta-bernoulli process
- gaussian process
- Markov Chain Monte Carlo
- Microscopy
- superresolution
- Fluorescence microscopy--Mathematical models.
- Fluorescence Microscopy
- Bayesian statistical decision theory--Scientific applications.
- Bayesian statistical decision theory
Resource Type
Collections this item is in
Note
- thesisPartial requirement for: M.A., Arizona State University, 2019
- bibliographyIncludes bibliographical references (pages 32-36)
- Field of study: Applied mathematics
Citation and reuse
Statement of Responsibility
by Ross Wallgren