Minimizing Dataset Size Requirements for Machine Learning
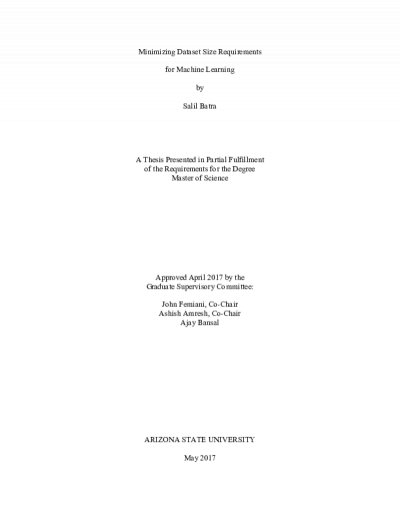
Description
Machine learning methodologies are widely used in almost all aspects of software engineering. An effective machine learning model requires large amounts of data to achieve high accuracy. The data used for classification is mostly labeled, which is difficult to obtain. The dataset requires both high costs and effort to accurately label the data into different classes. With abundance of data, it becomes necessary that all the data should be labeled for its proper utilization and this work focuses on reducing the labeling effort for large dataset. The thesis presents a comparison of different classifiers performance to test if small set of labeled data can be utilized to build accurate models for high prediction rate. The use of small dataset for classification is then extended to active machine learning methodology where, first a one class classifier will predict the outliers in the data and then the outlier samples are added to a training set for support vector machine classifier for labeling the unlabeled data. The labeling of dataset can be scaled up to avoid manual labeling and building more robust machine learning methodologies.
Date Created
The date the item was original created (prior to any relationship with the ASU Digital Repositories.)
2017
Agent
- Author (aut): Batra, Salil
- Thesis advisor (ths): Femiani, John
- Thesis advisor (ths): Amresh, Ashish
- Committee member: Bansal, Ajay
- Publisher (pbl): Arizona State University