Analysis Methods for No-Confounding Screening Designs
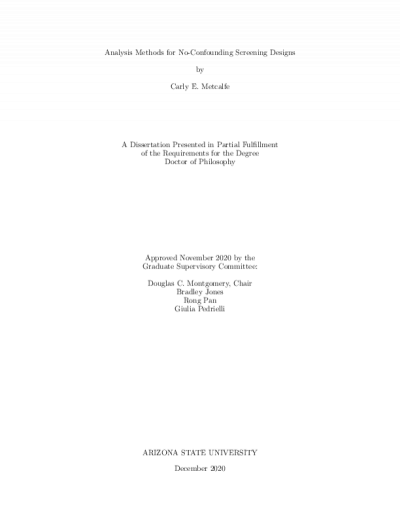
Description
Nonregular designs are a preferable alternative to regular resolution four designs because they avoid confounding two-factor interactions. As a result nonregular designs can estimate and identify a few active two-factor interactions. However, due to the sometimes complex alias structure of nonregular designs, standard screening strategies can fail to identify all active effects. In this research, two-level nonregular screening designs with orthogonal main effects will be discussed. By utilizing knowledge of the alias structure, a design based model selection process for analyzing nonregular designs is proposed.
The Aliased Informed Model Selection (AIMS) strategy is a design specific approach that is compared to three generic model selection methods; stepwise regression, least absolute shrinkage and selection operator (LASSO), and the Dantzig selector. The AIMS approach substantially increases the power to detect active main effects and two-factor interactions versus the aforementioned generic methodologies. This research identifies design specific model spaces; sets of models with strong heredity, all estimable, and exhibit no model confounding. These spaces are then used in the AIMS method along with design specific aliasing rules for model selection decisions. Model spaces and alias rules are identified for three designs; 16-run no-confounding 6, 7, and 8-factor designs. The designs are demonstrated with several examples as well as simulations to show the AIMS superiority in model selection.
A final piece of the research provides a method for augmenting no-confounding designs based on a model spaces and maximum average D-efficiency. Several augmented designs are provided for different situations. A final simulation with the augmented designs shows strong results for augmenting four additional runs if time and resources permit.
The Aliased Informed Model Selection (AIMS) strategy is a design specific approach that is compared to three generic model selection methods; stepwise regression, least absolute shrinkage and selection operator (LASSO), and the Dantzig selector. The AIMS approach substantially increases the power to detect active main effects and two-factor interactions versus the aforementioned generic methodologies. This research identifies design specific model spaces; sets of models with strong heredity, all estimable, and exhibit no model confounding. These spaces are then used in the AIMS method along with design specific aliasing rules for model selection decisions. Model spaces and alias rules are identified for three designs; 16-run no-confounding 6, 7, and 8-factor designs. The designs are demonstrated with several examples as well as simulations to show the AIMS superiority in model selection.
A final piece of the research provides a method for augmenting no-confounding designs based on a model spaces and maximum average D-efficiency. Several augmented designs are provided for different situations. A final simulation with the augmented designs shows strong results for augmenting four additional runs if time and resources permit.
Date Created
The date the item was original created (prior to any relationship with the ASU Digital Repositories.)
2020
Agent
- Author (aut): Metcalfe, Carly E
- Thesis advisor (ths): Montgomery, Douglas C.
- Committee member: Jones, Bradley
- Committee member: Pan, Rong
- Committee member: Pedrielli, Giulia
- Publisher (pbl): Arizona State University