System-level Models for Network Monitoring and Change Detection
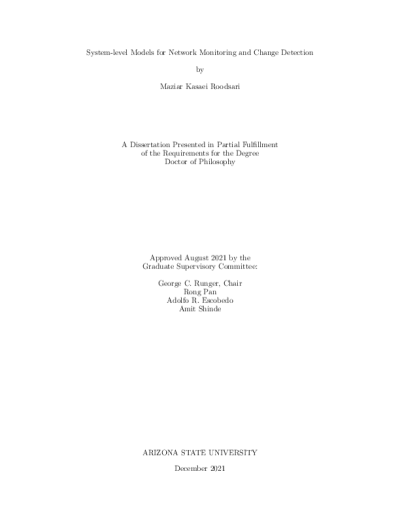
Description
Monitoring a system for deviations from standard or reference behavior is essential for many data-driven tasks. Whether it is monitoring sensor data or the interactions between system elements, such as edges in a path or transactions in a network, the goal is to detect significant changes from a reference. As technological advancements allow for more data to be collected from systems, monitoring approaches should evolve to accommodate the greater collection of high-dimensional data and complex system settings. This dissertation introduces system-level models for monitoring tasks characterized by changes in a subset of system components, utilizing component-level information and relationships. A change may only affect a portion of the data or system (partial change). The first three parts of this dissertation present applications and methods for detecting partial changes. The first part introduces a methodology for partial change detection in a simple, univariate setting. Changes are detected with posterior probabilities and statistical mixture models which allow only a fraction of data to change. The second and third parts of this dissertation center around monitoring more complex multivariate systems modeled through networks. The goal is to detect partial changes in the underlying network attributes and topology. The contributions of the second and third parts are two non-parametric system-level monitoring techniques that consider relationships between network elements. The algorithm Supervised Network Monitoring (SNetM) leverages Graph Neural Networks and transforms the problem into supervised learning. The other algorithm Supervised Network Monitoring for Partial Temporal Inhomogeneity (SNetMP) generates a network embedding, and then transforms the problem to supervised learning. At the end, both SNetM and SNetMP construct measures and transform them to pseudo-probabilities to be monitored for changes. The last topic addresses predicting and monitoring system-level delays on paths in a transportation/delivery system. For each item, the risk of delay is quantified. Machine learning is used to build a system-level model for delay risk, given the information available (such as environmental conditions) on the edges of a path, which integrates edge models. The outputs can then be used in a system-wide monitoring framework, and items most at risk are identified for potential corrective actions.
Date Created
The date the item was original created (prior to any relationship with the ASU Digital Repositories.)
2021
Agent
- Author (aut): Kasaei Roodsari, Maziar
- Thesis advisor (ths): Runger, George
- Committee member: Escobedo, Adolfo
- Committee member: Pan, Rong
- Committee member: Shinde, Amit
- Publisher (pbl): Arizona State University