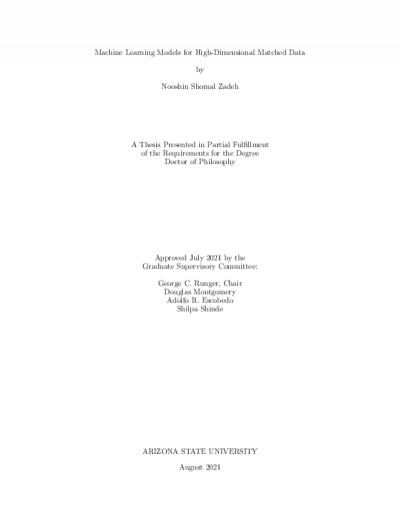
Description
Matching or stratification is commonly used in observational studies to remove bias due to confounding variables. Analyzing matched data sets requires specific methods which handle dependency among observations within a stratum. Also, modern studies often include hundreds or thousands of variables. Traditional methods for matched data sets are challenged in high-dimensional settings, mixed type variables (numerical and categorical), nonlinear andinteraction effects. Furthermore, machine learning research for such structured data is quite limited. This dissertation addresses this important gap and proposes machine learning models for identifying informative variables from high-dimensional matched data sets. The first part of this dissertation proposes a machine learning model to identify informative variables from high-dimensional matched case-control data sets. The outcome of interest in this study design is binary (case or control), and each stratum is assumed to have one unit from each outcome level. The proposed method which is referred to as Matched Forest (MF) is effective for large number of variables and identifying interaction effects. The second part of this dissertation proposes three enhancements of MF algorithm. First, a regularization framework is proposed to improve variable selection performance in excessively high-dimensional settings. Second, a classification method is proposed to classify unlabeled pairs of data. Third, two metrics are proposed to estimate the effects of important variables identified by MF. The third part proposes a machine learning model based on Neural Networks to identify important variables from a more generalized matched case-control data set where each stratum has one unit from case outcome level and more than one unit from control outcome level. This method which is referred to as Matched Neural Network (MNN) performs better than current algorithms to identify variables with interaction effects. Lastly, a generalized machine learning model is proposed to identify informative variables from high-dimensional matched data sets where the outcome has more than two levels. This method outperforms existing algorithms in the literature in identifying variables with complex nonlinear and interaction effects.
Download count: 3
Details
Title
- Machine Learning Models for High-Dimensional Matched Data
Contributors
- Shomal Zadeh, Nooshin (Author)
- Runger, George (Thesis advisor)
- Montgomery, Douglas (Committee member)
- Shinde, Shilpa (Committee member)
- Escobedo, Adolfo (Committee member)
- Arizona State University (Publisher)
Date Created
The date the item was original created (prior to any relationship with the ASU Digital Repositories.)
2021
Resource Type
Collections this item is in
Note
-
Partial requirement for: Ph.D., Arizona State University, 2021
-
Field of study: Industrial Engineering