Convoluted Processes: The Use and Misuse of Machine Learning in Data Analysis and Prediction
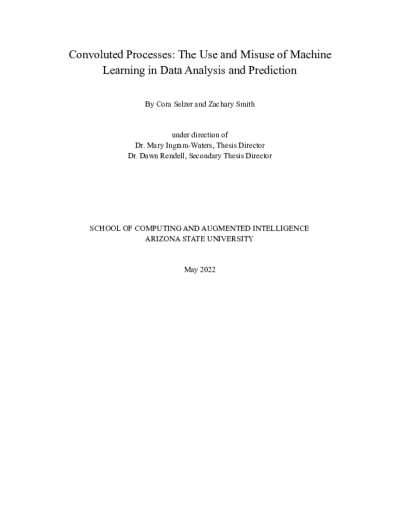
With the rapid increase of technological capabilities, particularly in processing power and speed, the usage of machine learning is becoming increasingly widespread, especially in fields where real-time assessment of complex data is extremely valuable. This surge in popularity of machine learning gives rise to an abundance of potential research and projects on further broadening applications of artificial intelligence. From these opportunities comes the purpose of this thesis. Our work seeks to meaningfully increase our understanding of current capabilities of machine learning and the problems they can solve. One extremely popular application of machine learning is in data prediction, as machines are capable of finding trends that humans often miss. Our effort to this end was to examine the CVE dataset and attempt to predict future entries with Random Forests. The second area of interest lies within the great promise being demonstrated by neural networks in the field of autonomous driving. We sought to understand the research being put out by the most prominent bodies within this field and to implement a model on one of the largest standing datasets, Berkeley DeepDrive 100k. This thesis describes our efforts to build, train, and optimize a Random Forest model on the CVE dataset and a convolutional neural network on the Berkeley DeepDrive 100k dataset. We document these efforts with the goal of growing our knowledge on (and usage of) machine learning in these topics.
- Author (aut): Selzer, Cora
- Co-author: Smith, Zachary
- Thesis director: Ingram-Waters, Mary
- Committee member: Rendell, Dawn
- Contributor (ctb): Barrett, The Honors College
- Contributor (ctb): Computer Science and Engineering Program
- Contributor (ctb): School of Mathematical and Statistical Sciences