Towards Reliable Semantic Vision
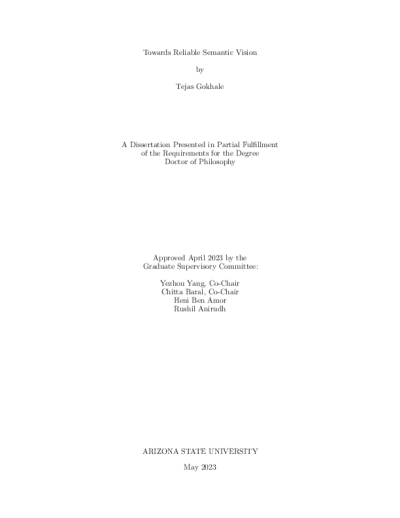
Description
Models that learn from data are widely and rapidly being deployed today for real-world use, and have become an integral and embedded part of human lives. While these technological advances are exciting and impactful, such data-driven computer vision systems often fail in inscrutable ways. This dissertation seeks to study and improve the reliability of machine learning models from several perspectives including the development of robust training algorithms to mitigate the risks of such failures, construction of new datasets that provide a new perspective on capabilities of vision models, and the design of evaluation metrics for re-calibrating the perception of performance improvements. I will first address distribution shift in image classification with the following contributions: (1) two methods for improving the robustness of image classifiers to distribution shift by leveraging the classifier's failures into an adversarial data transformation pipeline guided by domain knowledge, (2) an interpolation-based technique for flagging out-of-distribution samples, and (3) an intriguing trade-off between distributional and adversarial robustness resulting from data modification strategies. I will then explore reliability considerations for \textit{semantic vision} models that learn from both visual and natural language data; I will discuss how logical and semantic sentence transformations affect the performance of vision--language models and my contributions towards developing knowledge-guided learning algorithms to mitigate these failures. Finally, I will describe the effort towards building and evaluating complex reasoning capabilities of vision--language models towards the long-term goal of robust and reliable computer vision models that can communicate, collaborate, and reason with humans.
Date Created
The date the item was original created (prior to any relationship with the ASU Digital Repositories.)
2023
Agent
- Author (aut): Gokhale, Tejas
- Thesis advisor (ths): Yang, Yezhou
- Thesis advisor (ths): Baral, Chitta
- Committee member: Ben Amor, Heni
- Committee member: Anirudh, Rushil
- Publisher (pbl): Arizona State University