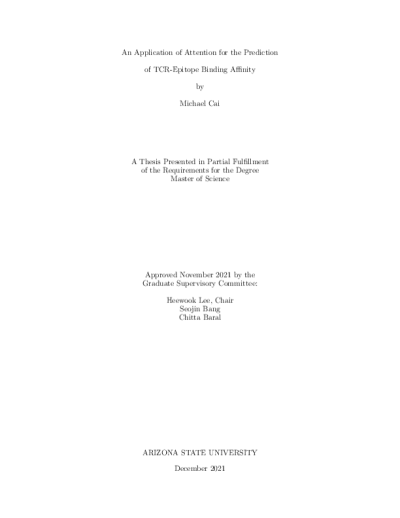
Description
T-cells are an integral component of the immune system, enabling the body to distinguish between pathogens and the self. The primary mechanism which enables this is their T-cell receptors (TCR) which bind to antigen epitopes foreign to the body. This detection mechanism allows the T-cell to determine when an immune response is necessary. The computational prediction of TCR-epitope binding is important to researchers for both medical applications and for furthering their understanding of the biological mechanisms that impact immunity. Models which have been developed for this purpose fail to account for the interrelationships between amino acids and demonstrate poor out-of-sample performance. Small changes to the amino acids in these protein sequences can drastically change their structure and function. In recent years, attention-based deep learning models have shown success in their ability to learn rich contextual representations of data. To capture the contextual biological relationships between the amino acids, a multi-head self-attention model was created to predict the binding affinity between given TCR and epitope sequences. By learning the structural nuances of the sequences, this model is able to improve upon existing model performance and grant insights into the underlying mechanisms which impact binding.
Details
Title
- An Application of Attention for the Prediction of TCR-Epitope Binding Affinity
Contributors
- Cai, Michael Ray (Author)
- Lee, Heewook (Thesis advisor)
- Bang, Seojin (Committee member)
- Baral, Chitta (Committee member)
- Arizona State University (Publisher)
Date Created
The date the item was original created (prior to any relationship with the ASU Digital Repositories.)
2021
Resource Type
Collections this item is in
Note
-
Partial requirement for: M.S., Arizona State University, 2021
-
Field of study: Computer Science