Uncertainty-Aware Neural Networks for Engineering Risk Assessment and Decision Support
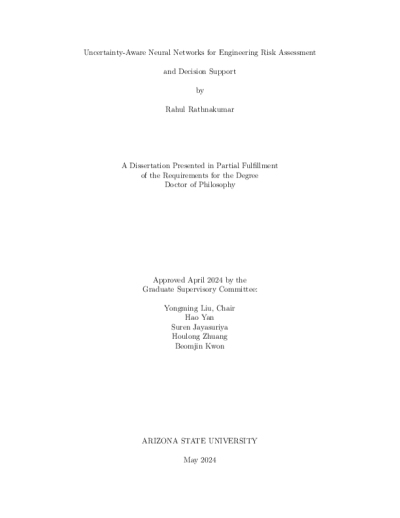
Description
This dissertation contributes to uncertainty-aware neural networks using multi-modality data, with a focus on industrial and aviation applications. Drawing from seminal works in recent years that have significantly advanced the field, this dissertation develops techniques for incorporating uncertainty estimation and leveraging multi-modality information into neural networks for tasks such as fault detection and environmental perception. The escalating complexity of data in engineering contexts demands models that predict accurately and quantify uncertainty in these predictions. The methods proposed in this document utilize various techniques, including Bayesian Deep Learning, multi-task regularization and feature fusion, and efficient use of unlabeled data. Popular methods of uncertainty quantification are analyzed empirically to derive important insights on their use in real world engineering problems. The primary objective is to develop and refine Bayesian neural network models for enhanced predictive accuracy and decision support in engineering. This involves exploring novel architectures, regularization methods, and data fusion techniques. Significant attention is given to data handling challenges in deep learning, particularly in the context of quality inspection systems. The research integrates deep learning with vision systems for engineering risk assessment and decision support tasks, and introduces two novel benchmark datasets designed for semantic segmentation and classification tasks. Additionally, the dissertation delves into RGB-Depth data fusion for pipeline defect detection and the use of semi-supervised learning algorithms for manufacturing inspection tasks with imaging data. The dissertation contributes to bridging the gap between advanced statistical methods and practical engineering applications.
Date Created
The date the item was original created (prior to any relationship with the ASU Digital Repositories.)
2024
Agent
- Author (aut): Rathnakumar, Rahul
- Thesis advisor (ths): Liu, Yongming
- Committee member: Yan, Hao
- Committee member: Jayasuriya, Suren
- Committee member: Zhuang, Houlong
- Committee member: Kwon, Beomjin
- Publisher (pbl): Arizona State University