Bridging the Physical and the Digital Worlds of Learning Analytics in Educational Assessments through Human-AI Collaboration
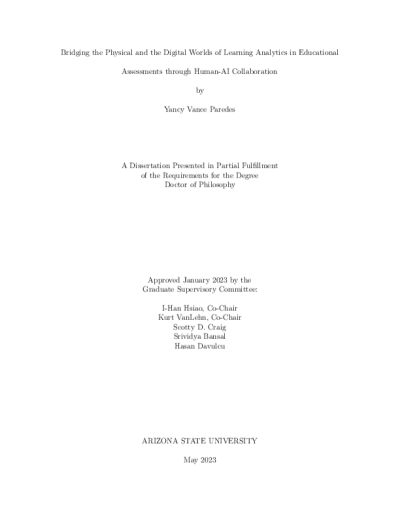
Description
Experience, whether personal or vicarious, plays an influential role in shaping human knowledge. Through these experiences, one develops an understanding of the world, which leads to learning. The process of gaining knowledge in higher education transcends beyond the passive transmission of knowledge from an expert to a novice. Instead, students are encouraged to actively engage in every learning opportunity to achieve mastery in their chosen field. Evaluation of such mastery typically entails using educational assessments that provide objective measures to determine whether the student has mastered what is required of them. With the proliferation of educational technology in the modern classroom, information about students is being collected at an unprecedented rate, covering demographic, performance, and behavioral data. In the absence of analytics expertise, stakeholders may miss out on valuable insights that can guide future instructional interventions, especially in helping students understand their strengths and weaknesses. This dissertation presents Web-Programming Grading Assistant (WebPGA), a homegrown educational technology designed based on various learning sciences principles, which has been used by 6,000+ students. In addition to streamlining and improving the grading process, it encourages students to reflect on their performance. WebPGA integrates learning analytics into educational assessments using students' physical and digital footprints. A series of classroom studies is presented demonstrating the use of learning analytics and assessment data to make students aware of their misconceptions. It aims to develop ways for students to learn from previous mistakes made by themselves or by others. The key findings of this dissertation include the identification of effective strategies of better-performing students, the demonstration of the importance of individualized guidance during the reviewing process, and the likely impact of validating one's understanding of another's experiences. Moreover, the Personalized Recommender of Items to Master and Evaluate (PRIME) framework is introduced. It is a novel and intelligent approach for diagnosing one's domain mastery and providing tailored learning opportunities by allowing students to observe others' mistakes. Thus, this dissertation lays the groundwork for further improvement and inspires better use of available data to improve the quality of educational assessments that will benefit both students and teachers.
Date Created
The date the item was original created (prior to any relationship with the ASU Digital Repositories.)
2023
Agent
- Author (aut): Paredes, Yancy Vance
- Thesis advisor (ths): Hsiao, I-Han
- Thesis advisor (ths): VanLehn, Kurt
- Committee member: Craig, Scotty D
- Committee member: Bansal, Srividya
- Committee member: Davulcu, Hasan
- Publisher (pbl): Arizona State University