Macronutrient Regulation by the Desert Leafcutter Ant Acromyrmex versicolor
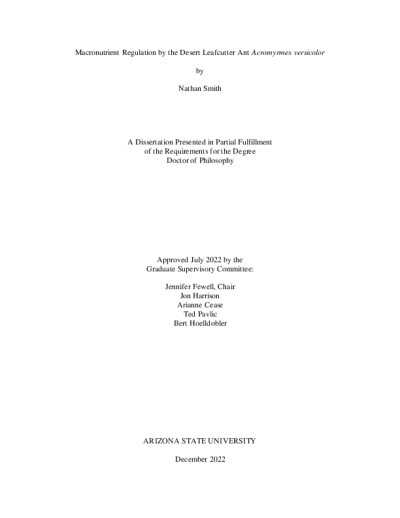
Description
Understanding how and why animals choose what to eat is one of the fundamental goals of nutritional and behavioral biology. This question can be scaled to animals that live in social groups, including eusocial insects. One of the factors that plays an important role in foraging decisions is the prevalence of specific nutrients and their relative balance. This dissertation explores the role of relative nutrient content in the food selection decisions of a species that is eusocial and also agricultural, the desert leafcutter ant Acromyrmex versicolor. A dietary choice assay, in which the relative amount of protein and carbohydrates in the available diets was varied, demonstrated that A. versicolor colonies regulate relative collection of protein and carbohydrates. Tracking the foraging behavior of individual workers revelaed that foragers vary in their relative collection of experimental diets and in their foraging frequency, but that there is no relationship between these key factors of foraging behavior. The high proportion of carbohydrates preferred by lab colonies suggests that they forage to nutritionally support the fungus rather than brood and workers. To test this, the relative amounts of 1) fungus, and 2) brood (larvae) was manipulated and foraging response was measured. Changing the amount of brood had no effect on foraging. Although decreasing the size of fungus gardens did not change relative P:C collection, it produced significant increases in caloric intake, supporting the assertion that the fungus is the main driver of colony nutrient regulation. The nutritional content of naturally harvested forage material collected from field colonies was measured, as was recruitment to experimental diets with varying relative macronutrient content. Field results confirmed a strong colony preference for high carbohydrate diets. They also indicated that this species may, at times, be limited in its ability to collect sufficiently high levels of carbohydrates to meet optimal intake. This dissertation provides important insights about fundamental aspects of leafcutter ant biology and extends our understanding of the role of relative nutrient content in foraging decisions to systems that span multiple trophic levels.
Date Created
The date the item was original created (prior to any relationship with the ASU Digital Repositories.)
2023
Agent
- Author (aut): Smith, Nathan Edward
- Thesis advisor (ths): Fewell, Jennifer H
- Committee member: Harrison, Jon F
- Committee member: Pavlic, Ted
- Committee member: Cease, Arianne
- Committee member: Hoelldobler, Bert
- Publisher (pbl): Arizona State University