Analysis and decision-making with social media
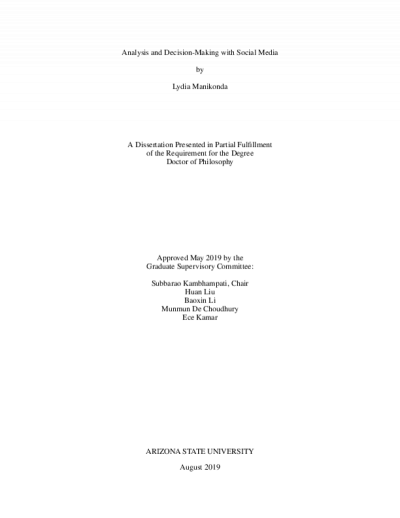
Description
The rapid advancements of technology have greatly extended the ubiquitous nature of smartphones acting as a gateway to numerous social media applications. This brings an immense convenience to the users of these applications wishing to stay connected to other individuals through sharing their statuses, posting their opinions, experiences, suggestions, etc on online social networks (OSNs). Exploring and analyzing this data has a great potential to enable deep and fine-grained insights into the behavior, emotions, and language of individuals in a society. This proposed dissertation focuses on utilizing these online social footprints to research two main threads – 1) Analysis: to study the behavior of individuals online (content analysis) and 2) Synthesis: to build models that influence the behavior of individuals offline (incomplete action models for decision-making).
A large percentage of posts shared online are in an unrestricted natural language format that is meant for human consumption. One of the demanding problems in this context is to leverage and develop approaches to automatically extract important insights from this incessant massive data pool. Efforts in this direction emphasize mining or extracting the wealth of latent information in the data from multiple OSNs independently. The first thread of this dissertation focuses on analytics to investigate the differentiated content-sharing behavior of individuals. The second thread of this dissertation attempts to build decision-making systems using social media data.
The results of the proposed dissertation emphasize the importance of considering multiple data types while interpreting the content shared on OSNs. They highlight the unique ways in which the data and the extracted patterns from text-based platforms or visual-based platforms complement and contrast in terms of their content. The proposed research demonstrated that, in many ways, the results obtained by focusing on either only text or only visual elements of content shared online could lead to biased insights. On the other hand, it also shows the power of a sequential set of patterns that have some sort of precedence relationships and collaboration between humans and automated planners.
A large percentage of posts shared online are in an unrestricted natural language format that is meant for human consumption. One of the demanding problems in this context is to leverage and develop approaches to automatically extract important insights from this incessant massive data pool. Efforts in this direction emphasize mining or extracting the wealth of latent information in the data from multiple OSNs independently. The first thread of this dissertation focuses on analytics to investigate the differentiated content-sharing behavior of individuals. The second thread of this dissertation attempts to build decision-making systems using social media data.
The results of the proposed dissertation emphasize the importance of considering multiple data types while interpreting the content shared on OSNs. They highlight the unique ways in which the data and the extracted patterns from text-based platforms or visual-based platforms complement and contrast in terms of their content. The proposed research demonstrated that, in many ways, the results obtained by focusing on either only text or only visual elements of content shared online could lead to biased insights. On the other hand, it also shows the power of a sequential set of patterns that have some sort of precedence relationships and collaboration between humans and automated planners.
Date Created
The date the item was original created (prior to any relationship with the ASU Digital Repositories.)
2019
Agent
- Author (aut): Manikonda, Lydia
- Thesis advisor (ths): Kambhampati, Subbarao
- Committee member: Liu, Huan
- Committee member: Li, Baoxin
- Committee member: De Choudhury, Munmun
- Committee member: Kamar, Ece
- Publisher (pbl): Arizona State University