Evaluating Social Influence in Health: Diabetes Assessments Among Latinos
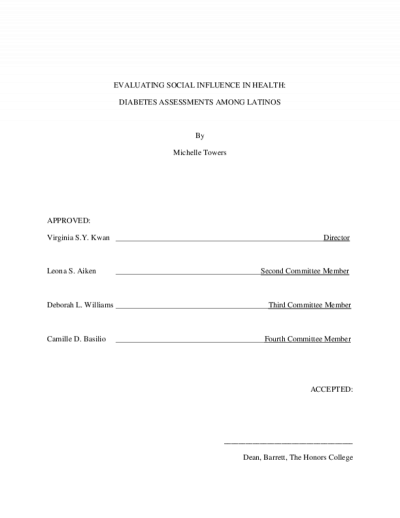
Description
Diabetes is prevalent among the Latino population in the United States. Engagement in healthy dietary behaviors, especially as a young adult, is an effective means of reducing risk for diabetes. Previous psychological theories have demonstrated that health beliefs and perceived barriers influence engagement in such behaviors. This research investigated beliefs regarding risk for diabetes among the young, educated Latino population. Study 1 of this research sought to compare health beliefs and perceived barriers to barrier change in the young, educated Latino and European American populations. Latinos reported to have a higher perceived vulnerability to diabetes, but shared the belief in diet as the most important determinant of diabetes risk with European Americans. However, Latinos saw their diet as less malleable in their lives than did European Americans. Study 2 sought to replicate these findings and verify the existence of these beliefs. Young, educated Latinos' beliefs in the importance of diet yet a perceived lack of dietary changeability were confirmed. Furthermore, Study 2 evaluated the efficacy of health messages based in the principle of social proof in motivating health behavior change. Social proof, or social validation, describes the phenomenon in which people who see others similar to them engaging in a particular behavior are more likely to engage in that behavior. Latinos who were randomly assigned to receive a health message utilizing the principle of social proof to motivate healthy dietary changes were more likely to express a willingness to change their diet than those who did not receive such a message. These findings can inform the development of health campaigns seeking to promote healthy behaviors among young, educated Latinos.
Date Created
The date the item was original created (prior to any relationship with the ASU Digital Repositories.)
2014-05
Agent
- Author (aut): Towers, Michelle
- Thesis director: Kwan, Virginia S. Y.
- Committee member: Aiken, Leona S.
- Committee member: Williams, Deborah L.
- Committee member: Basilio, Camille D.
- Contributor (ctb): Barrett, The Honors College