Examining Processes That Reflect Heterogeneity in Maritally Satisfied Couples
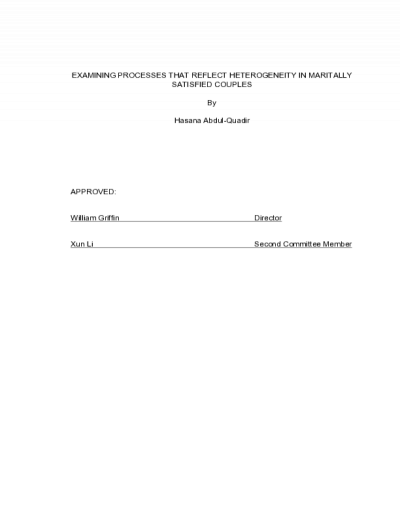
Description
In the mid-1970s, social scientists began observing marital dyad conversations in laboratory settings with the hope of determining which observable features best discriminate couples who report being either satisfied or unsatisfied with their relationship. These studies continued until about a decade ago when, in addition to increasing laboratory costs slowing the pace of new data collection, researchers realized that distressed couples were easier to quantitatively describe than nondistressed couples. Specifically, distressed couples exhibit rigid patterns of negativity whereas couples who report being maritally satisfied show minimal rigidity in the opposite direction \u2014 positivity. This was, and is, a theoretical dilemma: how can clinicians understand and eventually modify distressed relationships when the behavior of satisfied couples are less patterned, less predictable and more diverse? A recent study by Griffin and Li (2015), using contemporary machine learning techniques, reanalyzed existing marital interaction data and found that, contrary to expectation and existing theory, nondistressed couples should be further subdivided into two groups \u2014 those who are predictably positive or neutral and those who interact using diverse and varying levels of positive and negative behaviors. The latter group is the focus of this thesis. Using these recent findings as discussion points, I review how the unexpected behaviors in this novel group can maintain and possibly perpetuate marital satisfaction.
Date Created
The date the item was original created (prior to any relationship with the ASU Digital Repositories.)
2015-05
Agent
- Author (aut): Abdul-Quadir, Hasana
- Thesis director: Griffin, William A.
- Committee member: Li, Xun
- Contributor (ctb): Barrett, The Honors College
- Contributor (ctb): T. Denny Sanford School of Social and Family Dynamics
- Contributor (ctb): School of International Letters and Cultures
- Contributor (ctb): Hugh Downs School of Human Communication