Localizing Solar Power in Different Distribution Grid Feeders and Identification of the Meter-Transformer Connectivity
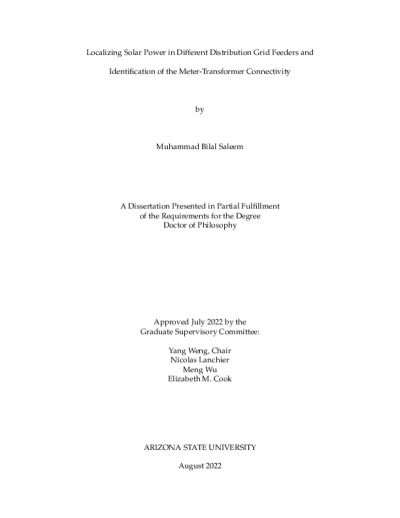
Description
The increase in the photovoltaic (PV) generation on distribution grids may cause reverse power flows and challenges such as service voltage violations and transformer overloading. To resolve these issues, utilities need situational awareness, e.g., PV-feeder mapping to identify the potential back-feeding feeders and meter-transformer mapping for transformer overloading. As circuit schematics are outdated, this work relies on data. In cases where the advanced metering infrastructure (AMI) data is unavailable, e.g., analog meters or bandwidth limitation, the dissertation proposes to use feeder measurements from utilities and solar panel measurements from solar companies to identify PV-feeder mapping. Several sequentially improved methods based on quantitative association rule mining (QARM) are proposed, where a lower bound for performance guarantee is also provided. However, binning data in QARM leads to information loss. So, bands are designed to replace bins for increased robustness. For cases where AMI data is available but solar PV data is unavailable, the AMI voltage data and location data are used for situational awareness, i.e., meter-transformer mapping, to resolve voltage violation and transformer overloading. A density-based clustering method is proposed that leverages AMI voltage data and geographical information to efficiently segment utility meters such that the segments comprise meters of few transformers only. Although it is helpful for utilities, it may not directly recover the meter-transformer connectivity, which requires transformer-wise segmentation. The proposed density-based method and other past methods ignore two common scenarios, e.g., having large distance between a meter and parent transformer or high similarity of a meter's consumption pattern to a non-parent transformer's meters. However, going from meter-meter can lead to the parent transformer group meters due to the usual observation that the similarity of intra-cluster meter voltages is usually stronger than the similarity of inter-cluster meter voltages. Therefore, performance guarantee is provided via spectral embedding with voltage data under reasonable assumption. Moreover, the assumption is partially relaxed using location data. It will benefit the utility in many ways, e.g., mitigating voltage violations by transformer tap settings and identifying overloaded transformers.
Date Created
The date the item was original created (prior to any relationship with the ASU Digital Repositories.)
2022
Agent
- Author (aut): Saleem, Muhammad Bilal
- Thesis advisor (ths): Weng, Yang
- Committee member: Lanchier, Nicolas
- Committee member: Wu, Meng
- Committee member: Cook, Elizabeth
- Publisher (pbl): Arizona State University