A Study on Generative Adversarial Networks Exacerbating Social Data Bias
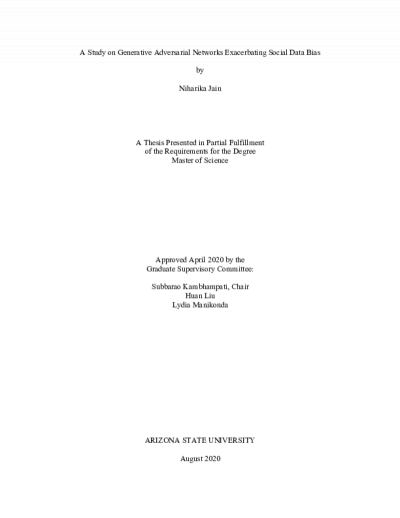
Description
Generative Adversarial Networks are designed, in theory, to replicate the distribution of the data they are trained on. With real-world limitations, such as finite network capacity and training set size, they inevitably suffer a yet unavoidable technical failure: mode collapse. GAN-generated data is not nearly as diverse as the real-world data the network is trained on; this work shows that this effect is especially drastic when the training data is highly non-uniform. Specifically, GANs learn to exacerbate the social biases which exist in the training set along sensitive axes such as gender and race. In an age where many datasets are curated from web and social media data (which are almost never balanced), this has dangerous implications for downstream tasks using GAN-generated synthetic data, such as data augmentation for classification. This thesis presents an empirical demonstration of this phenomenon and illustrates its real-world ramifications. It starts by showing that when asked to sample images from an illustrative dataset of engineering faculty headshots from 47 U.S. universities, unfortunately skewed toward white males, a DCGAN’s generator “imagines” faces with light skin colors and masculine features. In addition, this work verifies that the generated distribution diverges more from the real-world distribution when the training data is non-uniform than when it is uniform. This work also shows that a conditional variant of GAN is not immune to exacerbating sensitive social biases. Finally, this work contributes a preliminary case study on Snapchat’s explosively popular GAN-enabled “My Twin” selfie lens, which consistently lightens the skin tone for women of color in an attempt to make faces more feminine. The results and discussion of the study are meant to caution machine learning practitioners who may unsuspectingly increase the biases in their applications.
Date Created
The date the item was original created (prior to any relationship with the ASU Digital Repositories.)
2020
Agent
- Author (aut): Jain, Niharika
- Thesis advisor (ths): Kambhampati, Subbarao
- Committee member: Liu, Huan
- Committee member: Manikonda, Lydia
- Publisher (pbl): Arizona State University