Regression analysis of grouped counts and frequencies using the generalized linear model
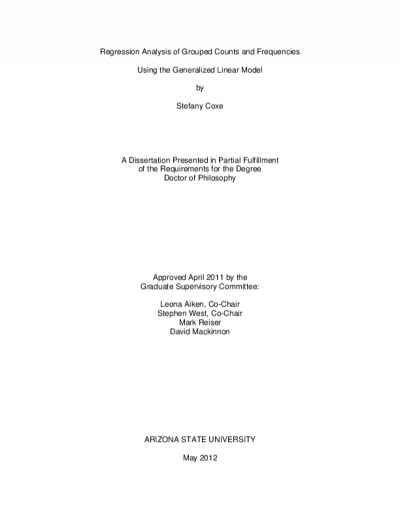
Description
Coarsely grouped counts or frequencies are commonly used in the behavioral sciences. Grouped count and grouped frequency (GCGF) that are used as outcome variables often violate the assumptions of linear regression as well as models designed for categorical outcomes; there is no analytic model that is designed specifically to accommodate GCGF outcomes. The purpose of this dissertation was to compare the statistical performance of four regression models (linear regression, Poisson regression, ordinal logistic regression, and beta regression) that can be used when the outcome is a GCGF variable. A simulation study was used to determine the power, type I error, and confidence interval (CI) coverage rates for these models under different conditions. Mean structure, variance structure, effect size, continuous or binary predictor, and sample size were included in the factorial design. Mean structures reflected either a linear relationship or an exponential relationship between the predictor and the outcome. Variance structures reflected homoscedastic (as in linear regression), heteroscedastic (monotonically increasing) or heteroscedastic (increasing then decreasing) variance. Small to medium, large, and very large effect sizes were examined. Sample sizes were 100, 200, 500, and 1000. Results of the simulation study showed that ordinal logistic regression produced type I error, statistical power, and CI coverage rates that were consistently within acceptable limits. Linear regression produced type I error and statistical power that were within acceptable limits, but CI coverage was too low for several conditions important to the analysis of counts and frequencies. Poisson regression and beta regression displayed inflated type I error, low statistical power, and low CI coverage rates for nearly all conditions. All models produced unbiased estimates of the regression coefficient. Based on the statistical performance of the four models, ordinal logistic regression seems to be the preferred method for analyzing GCGF outcomes. Linear regression also performed well, but CI coverage was too low for conditions with an exponential mean structure and/or heteroscedastic variance. Some aspects of model prediction, such as model fit, were not assessed here; more research is necessary to determine which statistical model best captures the unique properties of GCGF outcomes.
Date Created
The date the item was original created (prior to any relationship with the ASU Digital Repositories.)
2012
Agent
- Author (aut): Coxe, Stefany
- Thesis advisor (ths): Aiken, Leona S.
- Thesis advisor (ths): West, Stephen G.
- Committee member: Mackinnon, David P
- Committee member: Reiser, Mark R.
- Publisher (pbl): Arizona State University