Impact of Communities, Health, and Emotional-Related Factors on Smoking Use: Comparison of Joint Modeling of Mean and Dispersion and Bayes’ Hierarchical Models on Add Health Survey
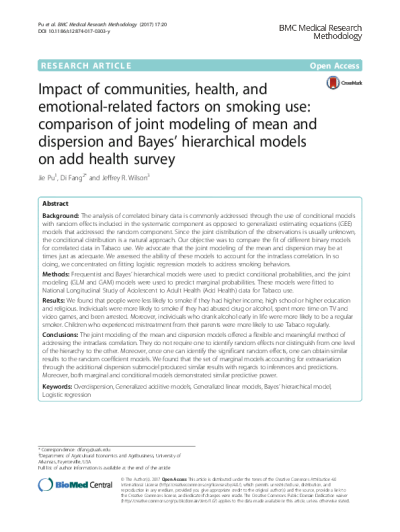
Background: The analysis of correlated binary data is commonly addressed through the use of conditional models with random effects included in the systematic component as opposed to generalized estimating equations (GEE) models that addressed the random component. Since the joint distribution of the observations is usually unknown, the conditional distribution is a natural approach. Our objective was to compare the fit of different binary models for correlated data in tobacco use. We advocate that the joint modeling of the mean and dispersion may be at times just as adequate. We assessed the ability of these models to account for the intraclass correlation. In so doing, we concentrated on fitting logistic regression models to address smoking behaviors.
Methods: Frequentist and Bayes’ hierarchical models were used to predict conditional probabilities, and the joint modeling (GLM and GAM) models were used to predict marginal probabilities. These models were fitted to National Longitudinal Study of Adolescent to Adult Health (Add Health) data for tobacco use.
Results: We found that people were less likely to smoke if they had higher income, high school or higher education and religious. Individuals were more likely to smoke if they had abused drug or alcohol, spent more time on TV and video games, and been arrested. Moreover, individuals who drank alcohol early in life were more likely to be a regular smoker. Children who experienced mistreatment from their parents were more likely to use tobacco regularly.
Conclusions: The joint modeling of the mean and dispersion models offered a flexible and meaningful method of addressing the intraclass correlation. They do not require one to identify random effects nor distinguish from one level of the hierarchy to the other. Moreover, once one can identify the significant random effects, one can obtain similar results to the random coefficient models. We found that the set of marginal models accounting for extravariation through the additional dispersion submodel produced similar results with regards to inferences and predictions. Moreover, both marginal and conditional models demonstrated similar predictive power.
- Author (aut): Pu, Jie
- Author (aut): Fang, Di
- Author (aut): Wilson, Jeffrey