Unobservable False Data Injection Attacks on Power Systems
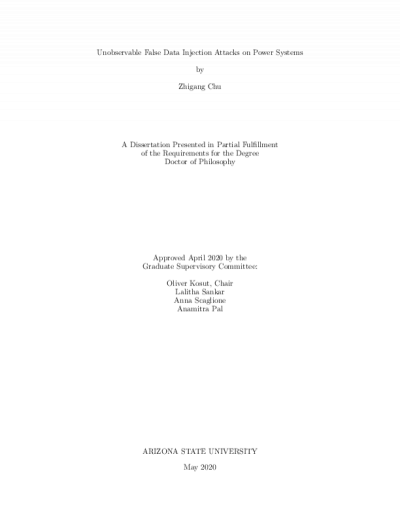
Description
Reliable operation of modern power systems is ensured by an intelligent cyber layer that monitors and controls the physical system. The data collection and transmission is achieved by the supervisory control and data acquisition (SCADA) system, and data processing is performed by the energy management system (EMS). In the recent decades, the development of phasor measurement units (PMUs) enables wide area real-time monitoring and control. However, both SCADA-based and PMU-based cyber layers are prone to cyber attacks that can impact system operation and lead to severe physical consequences.
This dissertation studies false data injection (FDI) attacks that are unobservable to bad data detectors (BDD). Prior work has shown that an attacker-defender bi-level linear program (ADBLP) can be used to determine the worst-case consequences of FDI attacks aiming to maximize the physical power flow on a target line. However, the results were only demonstrated on small systems assuming that they are operated with DC optimal power flow (OPF). This dissertation is divided into four parts to thoroughly understand the consequences of these attacks as well as develop countermeasures.
The first part focuses on evaluating the vulnerability of large-scale power systems to FDI attacks. The solution technique introduced in prior work to solve the ADBLP is intractable on large-scale systems due to the large number of binary variables. Four new computationally efficient algorithms are presented to solve this problem.
The second part studies vulnerability of N-1 reliable power systems operated by state-of-the-art EMSs commonly used in practice, specifically real-time contingency analysis (RTCA), and security-constrained economic dispatch (SCED). An ADBLP is formulated with detailed assumptions on attacker's knowledge and system operations.
The third part considers FDI attacks on PMU measurements that have strong temporal correlations due to high data rate. It is shown that predictive filters can detect suddenly injected attacks, but not gradually ramping attacks.
The last part proposes a machine learning-based attack detection framework consists of a support vector regression (SVR) load predictor that predicts loads by exploiting both spatial and temporal correlations, and a subsequent support vector machine (SVM) attack detector to determine the existence of attacks.
This dissertation studies false data injection (FDI) attacks that are unobservable to bad data detectors (BDD). Prior work has shown that an attacker-defender bi-level linear program (ADBLP) can be used to determine the worst-case consequences of FDI attacks aiming to maximize the physical power flow on a target line. However, the results were only demonstrated on small systems assuming that they are operated with DC optimal power flow (OPF). This dissertation is divided into four parts to thoroughly understand the consequences of these attacks as well as develop countermeasures.
The first part focuses on evaluating the vulnerability of large-scale power systems to FDI attacks. The solution technique introduced in prior work to solve the ADBLP is intractable on large-scale systems due to the large number of binary variables. Four new computationally efficient algorithms are presented to solve this problem.
The second part studies vulnerability of N-1 reliable power systems operated by state-of-the-art EMSs commonly used in practice, specifically real-time contingency analysis (RTCA), and security-constrained economic dispatch (SCED). An ADBLP is formulated with detailed assumptions on attacker's knowledge and system operations.
The third part considers FDI attacks on PMU measurements that have strong temporal correlations due to high data rate. It is shown that predictive filters can detect suddenly injected attacks, but not gradually ramping attacks.
The last part proposes a machine learning-based attack detection framework consists of a support vector regression (SVR) load predictor that predicts loads by exploiting both spatial and temporal correlations, and a subsequent support vector machine (SVM) attack detector to determine the existence of attacks.
Date Created
The date the item was original created (prior to any relationship with the ASU Digital Repositories.)
2020
Agent
- Author (aut): Chu, Zhigang
- Thesis advisor (ths): Kosut, Oliver
- Committee member: Sankar, Lalitha
- Committee member: Scaglione, Anna
- Committee member: Pal, Anamitra
- Publisher (pbl): Arizona State University