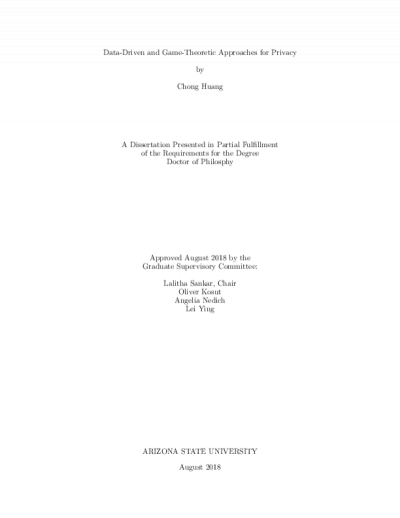
Description
In the past few decades, there has been a remarkable shift in the boundary between public and private information. The application of information technology and electronic communications allow service providers (businesses) to collect a large amount of data. However, this ``data collection" process can put the privacy of users at risk and also lead to user reluctance in accepting services or sharing data. This dissertation first investigates privacy sensitive consumer-retailers/service providers interactions under different scenarios, and then focuses on a unified framework for various information-theoretic privacy and privacy mechanisms that can be learned directly from data.
Existing approaches such as differential privacy or information-theoretic privacy try to quantify privacy risk but do not capture the subjective experience and heterogeneous expression of privacy-sensitivity. The first part of this dissertation introduces models to study consumer-retailer interaction problems and to better understand how retailers/service providers can balance their revenue objectives while being sensitive to user privacy concerns. This dissertation considers the following three scenarios: (i) the consumer-retailer interaction via personalized advertisements; (ii) incentive mechanisms that electrical utility providers need to offer for privacy sensitive consumers with alternative energy sources; (iii) the market viability of offering privacy guaranteed free online services. We use game-theoretic models to capture the behaviors of both consumers and retailers, and provide insights for retailers to maximize their profits when interacting with privacy sensitive consumers.
Preserving the utility of published datasets while simultaneously providing provable privacy guarantees is a well-known challenge. In the second part, a novel context-aware privacy framework called generative adversarial privacy (GAP) is introduced. Inspired by recent advancements in generative adversarial networks, GAP allows the data holder to learn the privatization mechanism directly from the data. Under GAP, finding the optimal privacy mechanism is formulated as a constrained minimax game between a privatizer and an adversary. For appropriately chosen adversarial loss functions, GAP provides privacy guarantees against strong information-theoretic adversaries. Both synthetic and real-world datasets are used to show that GAP can greatly reduce the adversary's capability of inferring private information at a small cost of distorting the data.
Existing approaches such as differential privacy or information-theoretic privacy try to quantify privacy risk but do not capture the subjective experience and heterogeneous expression of privacy-sensitivity. The first part of this dissertation introduces models to study consumer-retailer interaction problems and to better understand how retailers/service providers can balance their revenue objectives while being sensitive to user privacy concerns. This dissertation considers the following three scenarios: (i) the consumer-retailer interaction via personalized advertisements; (ii) incentive mechanisms that electrical utility providers need to offer for privacy sensitive consumers with alternative energy sources; (iii) the market viability of offering privacy guaranteed free online services. We use game-theoretic models to capture the behaviors of both consumers and retailers, and provide insights for retailers to maximize their profits when interacting with privacy sensitive consumers.
Preserving the utility of published datasets while simultaneously providing provable privacy guarantees is a well-known challenge. In the second part, a novel context-aware privacy framework called generative adversarial privacy (GAP) is introduced. Inspired by recent advancements in generative adversarial networks, GAP allows the data holder to learn the privatization mechanism directly from the data. Under GAP, finding the optimal privacy mechanism is formulated as a constrained minimax game between a privatizer and an adversary. For appropriately chosen adversarial loss functions, GAP provides privacy guarantees against strong information-theoretic adversaries. Both synthetic and real-world datasets are used to show that GAP can greatly reduce the adversary's capability of inferring private information at a small cost of distorting the data.
Details
Title
- Data-Driven and Game-Theoretic Approaches for Privacy
Contributors
- Huang, Chong (Author)
- Sankar, Lalitha (Thesis advisor)
- Kosut, Oliver (Committee member)
- Nedich, Angelia (Committee member)
- Ying, Lei (Committee member)
- Arizona State University (Publisher)
Date Created
The date the item was original created (prior to any relationship with the ASU Digital Repositories.)
2018
Subjects
Resource Type
Collections this item is in
Note
-
Doctoral Dissertation Electrical Engineering 2018