Quantifying Information Leakage via Adversarial Loss Functions: Theory and Practice
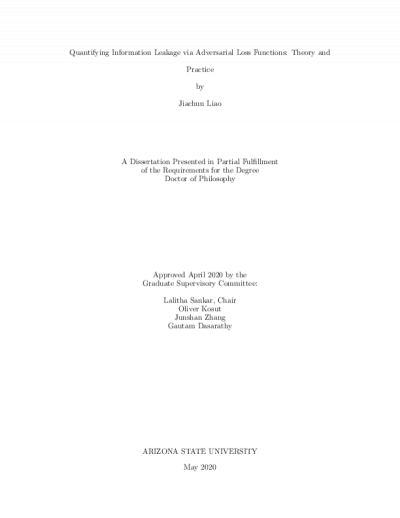
Description
Modern digital applications have significantly increased the leakage of private and sensitive personal data. While worst-case measures of leakage such as Differential Privacy (DP) provide the strongest guarantees, when utility matters, average-case information-theoretic measures can be more relevant. However, most such information-theoretic measures do not have clear operational meanings. This dissertation addresses this challenge.
This work introduces a tunable leakage measure called maximal $\alpha$-leakage which quantifies the maximal gain of an adversary in inferring any function of a data set. The inferential capability of the adversary is modeled by a class of loss functions, namely, $\alpha$-loss. The choice of $\alpha$ determines specific adversarial actions ranging from refining a belief for $\alpha =1$ to guessing the best posterior for $\alpha = \infty$, and for the two specific values maximal $\alpha$-leakage simplifies to mutual information and maximal leakage, respectively. Maximal $\alpha$-leakage is proved to have a composition property and be robust to side information.
There is a fundamental disjoint between theoretical measures of information leakages and their applications in practice. This issue is addressed in the second part of this dissertation by proposing a data-driven framework for learning Censored and Fair Universal Representations (CFUR) of data. This framework is formulated as a constrained minimax optimization of the expected $\alpha$-loss where the constraint ensures a measure of the usefulness of the representation. The performance of the CFUR framework with $\alpha=1$ is evaluated on publicly accessible data sets; it is shown that multiple sensitive features can be effectively censored to achieve group fairness via demographic parity while ensuring accuracy for several \textit{a priori} unknown downstream tasks.
Finally, focusing on worst-case measures, novel information-theoretic tools are used to refine the existing relationship between two such measures, $(\epsilon,\delta)$-DP and R\'enyi-DP. Applying these tools to the moments accountant framework, one can track the privacy guarantee achieved by adding Gaussian noise to Stochastic Gradient Descent (SGD) algorithms. Relative to state-of-the-art, for the same privacy budget, this method allows about 100 more SGD rounds for training deep learning models.
This work introduces a tunable leakage measure called maximal $\alpha$-leakage which quantifies the maximal gain of an adversary in inferring any function of a data set. The inferential capability of the adversary is modeled by a class of loss functions, namely, $\alpha$-loss. The choice of $\alpha$ determines specific adversarial actions ranging from refining a belief for $\alpha =1$ to guessing the best posterior for $\alpha = \infty$, and for the two specific values maximal $\alpha$-leakage simplifies to mutual information and maximal leakage, respectively. Maximal $\alpha$-leakage is proved to have a composition property and be robust to side information.
There is a fundamental disjoint between theoretical measures of information leakages and their applications in practice. This issue is addressed in the second part of this dissertation by proposing a data-driven framework for learning Censored and Fair Universal Representations (CFUR) of data. This framework is formulated as a constrained minimax optimization of the expected $\alpha$-loss where the constraint ensures a measure of the usefulness of the representation. The performance of the CFUR framework with $\alpha=1$ is evaluated on publicly accessible data sets; it is shown that multiple sensitive features can be effectively censored to achieve group fairness via demographic parity while ensuring accuracy for several \textit{a priori} unknown downstream tasks.
Finally, focusing on worst-case measures, novel information-theoretic tools are used to refine the existing relationship between two such measures, $(\epsilon,\delta)$-DP and R\'enyi-DP. Applying these tools to the moments accountant framework, one can track the privacy guarantee achieved by adding Gaussian noise to Stochastic Gradient Descent (SGD) algorithms. Relative to state-of-the-art, for the same privacy budget, this method allows about 100 more SGD rounds for training deep learning models.
Date Created
The date the item was original created (prior to any relationship with the ASU Digital Repositories.)
2020
Agent
- Author (aut): Liao, Jiachun
- Thesis advisor (ths): Sankar, Lalitha
- Committee member: Kosut, Oliver
- Committee member: Zhang, Junshan
- Committee member: Dasarathy, Gautam
- Publisher (pbl): Arizona State University