Determining the Feasibility of Statistical Techniques to Identify the Most Important Input Parameters of Building Energy Models
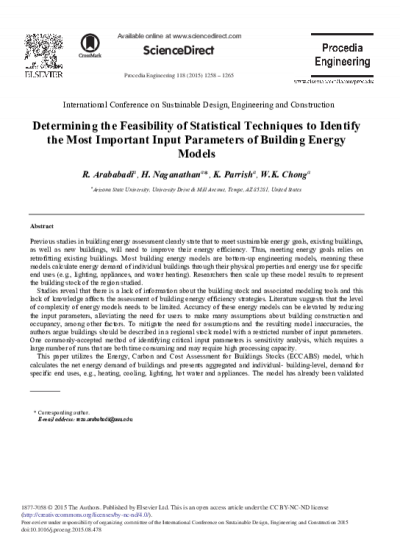
Previous studies in building energy assessment clearly state that to meet sustainable energy goals, existing buildings, as well as new buildings, will need to improve their energy efficiency. Thus, meeting energy goals relies on retrofitting existing buildings. Most building energy models are bottom-up engineering models, meaning these models calculate energy demand of individual buildings through their physical properties and energy use for specific end uses (e.g., lighting, appliances, and water heating). Researchers then scale up these model results to represent the building stock of the region studied.
Studies reveal that there is a lack of information about the building stock and associated modeling tools and this lack of knowledge affects the assessment of building energy efficiency strategies. Literature suggests that the level of complexity of energy models needs to be limited. Accuracy of these energy models can be elevated by reducing the input parameters, alleviating the need for users to make many assumptions about building construction and occupancy, among other factors. To mitigate the need for assumptions and the resulting model inaccuracies, the authors argue buildings should be described in a regional stock model with a restricted number of input parameters. One commonly-accepted method of identifying critical input parameters is sensitivity analysis, which requires a large number of runs that are both time consuming and may require high processing capacity.
This paper utilizes the Energy, Carbon and Cost Assessment for Buildings Stocks (ECCABS) model, which calculates the net energy demand of buildings and presents aggregated and individual- building-level, demand for specific end uses, e.g., heating, cooling, lighting, hot water and appliances. The model has already been validated using the Swedish, Spanish, and UK building stock data. This paper discusses potential improvements to this model by assessing the feasibility of using stepwise regression to identify the most important input parameters using the data from UK residential sector. The paper presents results of stepwise regression and compares these to sensitivity analysis; finally, the paper documents the advantages and challenges associated with each method.
- Author (aut): Arababadi, Reza
- Author (aut): Naganathan, Hariharan
- Author (aut): Parrish, Kristen
- Author (aut): Chong, Oswald
- Contributor (ctb): Ira A. Fulton Schools of Engineering