Characterization and Optimization of ReRAM-based Analog Crossbar Arrays for Neuromorphic Computing
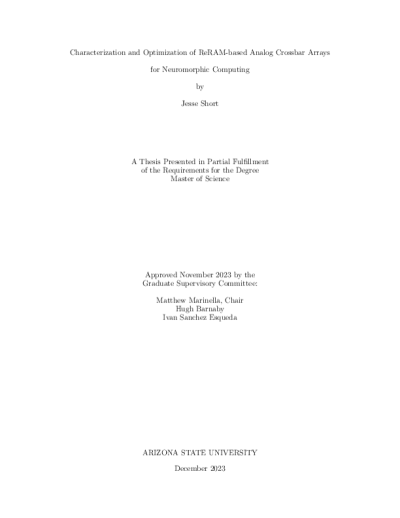
Description
Machine learning advancements have led to increasingly complex algorithms, resulting in significant energy consumption due to heightened memory-transfer requirements and inefficient vector matrix multiplication (VMM). To address this issue, many have proposed ReRAM analog in-memory computing (AIMC) as a solution. AIMC enhances the time-energy efficiency of VMM operations beyond conventional VMM digital hardware, such as a tensor processing unit (TPU), while substantially reducing memory-transfer demands through in-memory computing. As AIMC gains prominence as a solution, it becomes crucial to optimize ReRAM and analog crossbar architecture characteristics. This thesis introduces an application-specific integrated circuit (ASIC) tailored forcharacterizing ReRAM within a crossbar array architecture and discusses the interfacing techniques employed. It discusses ReRAM forming and programming techniques and showcases chip’s ability to utilize the write-verify programming method to write image pixels on a conductance heat map. Additionally, this thesis assesses the ASIC’s capability to characterize different aspects of ReRAM, including drift and noise characteristics. The research employs the chip to extract ReRAM data and models it within a crossbar array simulator, enabling its application in the classification of the CIFAR-10 dataset.
Date Created
The date the item was original created (prior to any relationship with the ASU Digital Repositories.)
2023
Agent
- Author (aut): Short, Jesse
- Thesis advisor (ths): Marinella, Matthew
- Committee member: Barnaby, Hugh
- Committee member: Sanchez Esqueda, Ivan
- Publisher (pbl): Arizona State University