Towards Very Early Interrogation of Neurodegenerative Diseases with Diffusion MRI
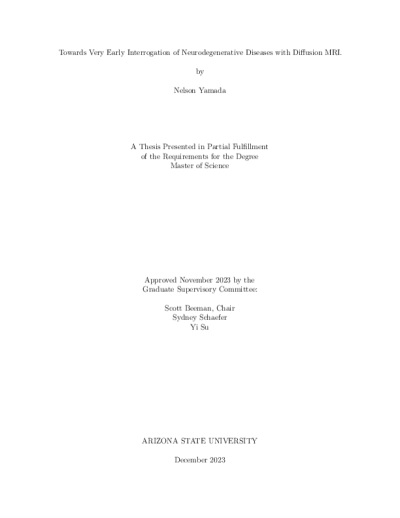
Description
It is hypothesized that changes in brain tissue microstructure, particularly degradation of neurites (i.e,. axons and dendrites) and synapses, are early drivers of Alzheimer's disease (AD) pathogenesis. Quantitative magnetic resonance imaging (MRI) tools like diffusion tensor imaging (DTI) have long been used to study AD pathogenesis. Using DTI metrics, structural insights of neuro tissue can be inferred but not directly measured. DTI has proven to be an effective tool indicating fractional anisotrophy (FA) differences between groups of varying AD risk factor, but it does not explicitly quantify pathophysiologically-relevant features like neurite density and complexity. This study aims to develop and validate an advanced diffusion MRI acquisition and biophysical modeling platform that can be used to explicitly quantify changes to brain tissue microstructure, specifically neurite density and complexity. Ultimately, this platform will be used to study the pathogenic mechanisms that drive AD in the pre-clinical and clinical setting.
Date Created
The date the item was original created (prior to any relationship with the ASU Digital Repositories.)
2023
Agent
- Author (aut): Yamada, Nelson Garr
- Thesis advisor (ths): Beeman, Scott
- Committee member: Schaefer, Sydney
- Committee member: Su, Yi
- Publisher (pbl): Arizona State University