Use of Machine Learning and Data Science on Infrastructure Transportation and Construction Projects
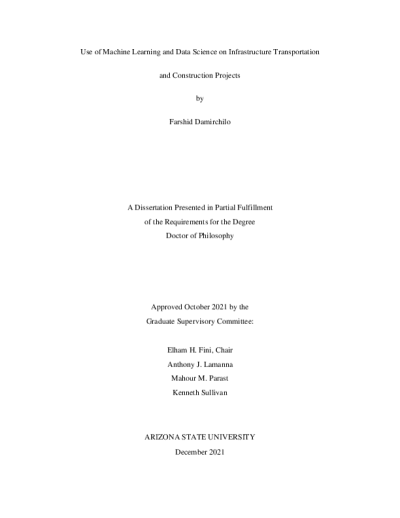
Description
By the evolution of technologies and computing power, it is possible to capture and save large amounts of data and then find patterns in large and complex datasets using data science and machine learning. This dissertation introduces machine-learning models and econometric models to use in infrastructure transportation projects. Among transportation infrastructure projects, the airline industry and highways are selected to implement the models.The first topic of this dissertation focuses on using machine-learning models in highway projects. The International Roughness Index (IRI) for asphalt concrete pavement is predicted based on the 12,637 observations in the Long-Term Pavement Performance (LTPP) dataset for 1,390 roads and highways in the 50 states of the United States and the District of Columbia from 1989 to 2018. The results show that XGBoost provides a better model fit in terms of mean absolute error and coefficient of determination than other studied models. Also, the most important factors in predicting the IRI are identified.
The second topic of this dissertation aims to develop machine-learning models to predict customer dissatisfaction in the airline industry. The relationship between measures of service failure (flight delay and mishandled baggage) and customer dissatisfaction is predicted by using longitudinal data from 2003 to 2019 from the U.S. airline industry. Data was obtained from the Air Travel Consumer Report (ATCR) published by the U.S. Department of Transportation. Flight delay is more important in low-cost airlines, while mishandled baggage is more important in legacy airlines. Also, the effect of the train-test split ratio on each machine-learning model is examined by running each model using four train-test splits. Results indicate that the train-test split ratio could influence the selection of the best model.
The third topic in this dissertation uses econometric analysis to investigate the relationship between customer dissatisfaction and two measures of service failure in the U.S. airline industry. Results are: 1) Mishandled baggage has more impact than flight delay on customer complaints. 2) The effect of an airline’s service failures on customer complaints is contingent on the category of the airline. 3) The effect of flight delay on customer complaints is lower for low-cost airlines compared to legacy airlines.
Date Created
The date the item was original created (prior to any relationship with the ASU Digital Repositories.)
2021
Agent
- Author (aut): Damirchilo, Farshid
- Thesis advisor (ths): Fini, Elham H
- Committee member: Lamanna, Anthony J
- Committee member: Parast, Mahour M
- Committee member: Sullivan, Kenneth
- Publisher (pbl): Arizona State University