Hacking the Learning Curve: Effective Cybersecurity Education at Scale
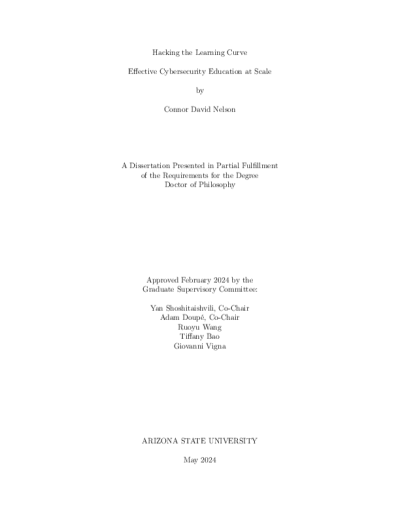
Description
This dissertation introduces a comprehensive framework aimed at reshaping applied cybersecurity education to significantly ease the learning curve, at scale, through three synergistic innovations. These methods address the daunting educational barriers in cybersecurity, enabling learners at all levels to understand complex security concepts more easily. The first innovation, the PWN methodology, redefines the traditional Capture The Flag (CTF) model by offering a structured series of modularized, self-guided challenges. This approach helps simplify complex topics into manageable units, each building on the last, which allows students to progress at their own pace. Over five years and with over 400 systems security challenges developed, this method has effectively helped students evolve from beginners to masters of advanced security exploits. The second component is the DOJO platform, an open-source learning environment that uses containerization technology to provide a pre-configured, browser-based interface. This platform reduces the setup complexities associated with applied cybersecurity and has already given over 10,000 students immediate access to practical learning scenarios, from vulnerability discovery to advanced debugging, in a unified, user-friendly environment. Its seamless integration allows educators to quickly launch new challenges and resources, ensuring a continuous and dynamic educational experience. The third component, the SENSAI tutor, is an AI-driven tutoring system that leverages Large Language Models to offer personalized, intelligent support. Integrated with the PWN methodology and DOJO platform, SENSAI serves as an on-demand mentor, providing tailored advice and problem-solving assistance. It adapts to individual student needs, offering specific guidance and theoretical support to enhance understanding and retention of complex concepts. Together, these three components create a powerful, integrated educational strategy that not only equips students with vital cybersecurity skills but also deepens their understanding of digital vulnerabilities and the strategic thinking needed to mitigate them. This strategy prepares a new generation of cybersecurity professionals to navigate the ever-evolving threats of the digital world.
Date Created
The date the item was original created (prior to any relationship with the ASU Digital Repositories.)
2024
Agent
- Author (aut): Nelson, Connor David
- Thesis advisor (ths): Shoshitaishvili, Yan
- Thesis advisor (ths): Doupe, Adam
- Committee member: Wang, Ruoyu
- Committee member: Bao, Tiffany
- Committee member: Vigna, Giovanni
- Publisher (pbl): Arizona State University