Energy Management System in Naval Submarines
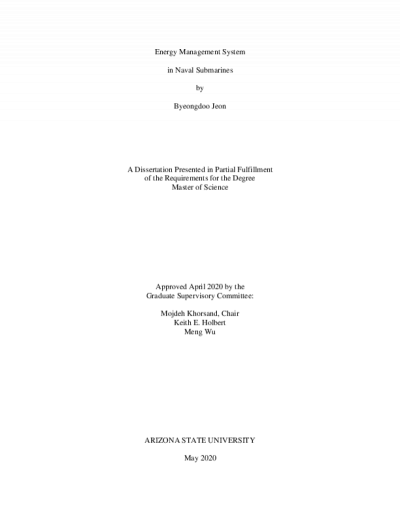
Description
An optimal energy scheduling procedure is essential in an isolated environment such as naval submarines. Conventional naval submarines include diesel-electric propulsion systems, which utilize diesel generators along with batteries and fuel cells. Submarines can charge the batteries by running diesel-electric generators only at the surface or at snorkeling depth. This is the most dangerous time for submarines to be detectable by acoustic and non-acoustic sensors of enemy assets. Optimizing the energy resources while reducing the need for snorkeling is the main factor to enhance underwater endurance. This thesis introduces an energy management system (EMS) as a supervisory tool for the officers onboard to plan energy schedules in order to complete various missions. The EMS for a 4,000-ton class conventional submarine is developed to minimize snorkeling and satisfy various conditions of practically designed missions by optimizing the energy resources, such as Lithium-ion batteries, Proton-exchange membrane fuel cells, and diesel-electric generators. Eventually, the optimized energy schedules with the minimum snorkeling hours are produced for five mission scenarios. More importantly, this EMS performs deterministic and stochastic operational scheduling processes to provide secured optimal schedules which contains outages in the power generation and storage systems.
Date Created
The date the item was original created (prior to any relationship with the ASU Digital Repositories.)
2020
Agent
- Author (aut): Jeon, Byeongdoo
- Thesis advisor (ths): Hedman, Mojdeh Khorsand
- Committee member: Holbert, Keith E.
- Committee member: Wu, Meng
- Publisher (pbl): Arizona State University