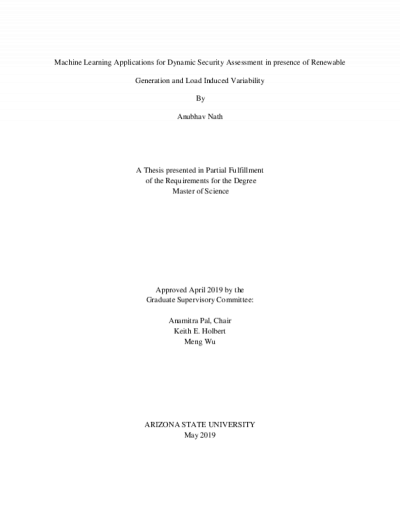
Description
Large-scale blackouts that have occurred across North America in the past few decades have paved the path for substantial amount of research in the field of security assessment of the grid. With the aid of advanced technology such as phasor measurement units (PMUs), considerable work has been done involving voltage stability analysis and power system dynamic behavior analysis to ensure security and reliability of the grid. Online dynamic security assessment (DSA) analysis has been developed and applied in several power system control centers. Existing applications of DSA are limited by the assumption of simplistic load profiles, which often considers a normative day to represent an entire year. To overcome these aforementioned challenges, this research developed a novel DSA scheme to provide security prediction in real-time for load profiles corresponding to different seasons. The major contributions of this research are to (1) develop a DSA scheme incorporated with PMU data, (2) consider a comprehensive seasonal load profile, (3) account for varying penetrations of renewable generation, and (4) compare the accuracy of different machine learning (ML) algorithms for DSA. The ML algorithms that will be the focus of this study include decision trees (DTs), support vector machines (SVMs), random forests (RFs), and multilayer neural networks (MLNNs).
This thesis describes the development of a novel DSA scheme using synchrophasor measurements that accounts for the load variability occurring across different seasons in a year. Different amounts of solar generation have also been incorporated in this study to account for increasing percentage of renewables in the modern grid. To account for the security of the operating conditions different ML algorithms have been trained and tested. A database of cases for different operating conditions has been developed offline that contains secure as well as insecure cases, and the ML models have been trained to classify the security or insecurity of a particular operating condition in real-time. Multiple scenarios are generated every 15 minutes for different seasons and stored in the database. The performance of this approach is tested on the IEEE-118 bus system.
This thesis describes the development of a novel DSA scheme using synchrophasor measurements that accounts for the load variability occurring across different seasons in a year. Different amounts of solar generation have also been incorporated in this study to account for increasing percentage of renewables in the modern grid. To account for the security of the operating conditions different ML algorithms have been trained and tested. A database of cases for different operating conditions has been developed offline that contains secure as well as insecure cases, and the ML models have been trained to classify the security or insecurity of a particular operating condition in real-time. Multiple scenarios are generated every 15 minutes for different seasons and stored in the database. The performance of this approach is tested on the IEEE-118 bus system.
Details
Title
- Machine Learning Applications for Dynamic Security Assessment in presence of Renewable Generation and Load Induced Variability
Contributors
- NATH, ANUBHAV (Author)
- Pal, Anamitra (Thesis advisor)
- Holbert, Keith (Committee member)
- Wu, Meng (Committee member)
- Arizona State University (Publisher)
Date Created
The date the item was original created (prior to any relationship with the ASU Digital Repositories.)
2019
Subjects
Resource Type
Collections this item is in
Note
- Masters Thesis Electrical Engineering 2019