An Analysis of the Boundary Explorer Adaptive Sampling Technique
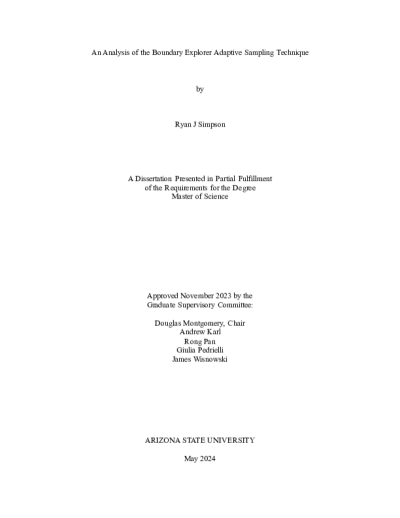
Description
With the explosion of autonomous systems under development, complex simulation models are being tested and relied on far more than in the recent past. This uptick in autonomous systems being modeled then tested magnifies both the advantages and disadvantages of simulation experimentation. An inherent problem in autonomous systems development is when small changes in factor settings result in large changes in a response’s performance. These occurrences look like cliffs in a metamodel’s response surface and are referred to as performance mode boundary regions. These regions represent areas of interest in the autonomous system’s decision-making process. Therefore, performance mode boundary regions are areas of interest for autonomous systems developers.Traditional augmentation methods aid experimenters seeking different objectives, often by improving a certain design property of the factor space (such as variance) or a design’s modeling capabilities. While useful, these augmentation techniques do not target areas of interest that need attention in autonomous systems testing focused on the response. Boundary Explorer Adaptive Sampling Technique, or BEAST, is a set of design augmentation algorithms. The adaptive sampling algorithm targets performance mode boundaries with additional samples. The gap filling augmentation algorithm targets sparsely sampled areas in the factor space. BEAST allows for sampling to adapt to information obtained from pervious iterations of experimentation and target these regions of interest. Exploiting the advantages of simulation model experimentation, BEAST can be used to provide additional iterations of experimentation, providing clarity and high-fidelity in areas of interest along potentially steep gradient regions.
The objective of this thesis is to research and present BEAST, then compare BEAST’s algorithms to other design augmentation techniques. Comparisons are made towards traditional methods that are already implemented in SAS Institute’s JMP software, or emerging adaptive sampling techniques, such as Range Adversarial Planning Tool (RAPT). The goal of this objective is to gain a deeper understanding of how BEAST works and where it stands in the design augmentation space for practical applications. With a gained understanding of how BEAST operates and how well BEAST performs, future research recommendations will be presented to improve BEAST’s capabilities.
Date Created
The date the item was original created (prior to any relationship with the ASU Digital Repositories.)
2024
Agent
- Author (aut): Simpson, Ryan James
- Thesis advisor (ths): Montgomery, Douglas
- Committee member: Karl, Andrew
- Committee member: Pan, Rong
- Committee member: Pedrielli, Giulia
- Committee member: Wisnowski, James
- Publisher (pbl): Arizona State University