Hash Families and Applications to t-Restrictions
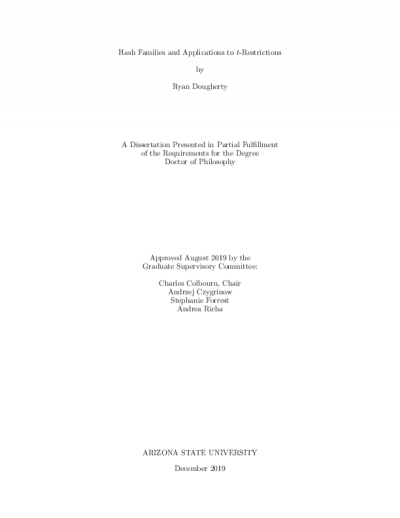
Description
The construction of many families of combinatorial objects remains a challenging problem. A t-restriction is an array where a predicate is satisfied for every t columns; an example is a perfect hash family (PHF). The composition of a PHF and any t-restriction satisfying predicate P yields another t-restriction also satisfying P with more columns than the original t-restriction had. This thesis concerns three approaches in determining the smallest size of PHFs.
Firstly, hash families in which the associated property is satisfied at least some number lambda times are considered, called higher-index, which guarantees redundancy when constructing t-restrictions. Some direct and optimal constructions of hash families of higher index are given. A new recursive construction is established that generalizes previous results and generates higher-index PHFs with more columns. Probabilistic methods are employed to obtain an upper bound on the optimal size of higher-index PHFs when the number of columns is large. A new deterministic algorithm is developed that generates such PHFs meeting this bound, and computational results are reported.
Secondly, a restriction on the structure of PHFs is introduced, called fractal, a method from Blackburn. His method is extended in several ways; from homogeneous hash families (every row has the same number of symbols) to heterogeneous ones; and to distributing hash families, a relaxation of the predicate for PHFs. Recursive constructions with fractal hash families as ingredients are given, and improve upon on the best-known sizes of many PHFs.
Thirdly, a method of Colbourn and Lanus is extended in which they horizontally copied a given hash family and greedily applied transformations to each copy. Transformations of existential t-restrictions are introduced, which allow for the method to be applicable to any t-restriction having structure like those of hash families. A genetic algorithm is employed for finding the "best" such transformations. Computational results of the GA are reported using PHFs, as the number of transformations permitted is large compared to the number of symbols. Finally, an analysis is given of what trade-offs exist between computation time and the number of t-sets left not satisfying the predicate.
Firstly, hash families in which the associated property is satisfied at least some number lambda times are considered, called higher-index, which guarantees redundancy when constructing t-restrictions. Some direct and optimal constructions of hash families of higher index are given. A new recursive construction is established that generalizes previous results and generates higher-index PHFs with more columns. Probabilistic methods are employed to obtain an upper bound on the optimal size of higher-index PHFs when the number of columns is large. A new deterministic algorithm is developed that generates such PHFs meeting this bound, and computational results are reported.
Secondly, a restriction on the structure of PHFs is introduced, called fractal, a method from Blackburn. His method is extended in several ways; from homogeneous hash families (every row has the same number of symbols) to heterogeneous ones; and to distributing hash families, a relaxation of the predicate for PHFs. Recursive constructions with fractal hash families as ingredients are given, and improve upon on the best-known sizes of many PHFs.
Thirdly, a method of Colbourn and Lanus is extended in which they horizontally copied a given hash family and greedily applied transformations to each copy. Transformations of existential t-restrictions are introduced, which allow for the method to be applicable to any t-restriction having structure like those of hash families. A genetic algorithm is employed for finding the "best" such transformations. Computational results of the GA are reported using PHFs, as the number of transformations permitted is large compared to the number of symbols. Finally, an analysis is given of what trade-offs exist between computation time and the number of t-sets left not satisfying the predicate.
Date Created
The date the item was original created (prior to any relationship with the ASU Digital Repositories.)
2019
Agent
- Author (aut): Dougherty, Ryan Edward
- Thesis advisor (ths): Colbourn, Charles J
- Committee member: Czygrinow, Andrzej
- Committee member: Forrest, Stephanie
- Committee member: Richa, Andrea
- Publisher (pbl): Arizona State University