Downsampling for Efficient Parameter Choice in Ill-Posed Deconvolution Problems
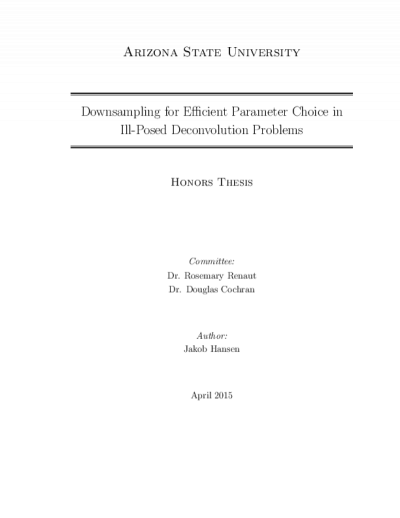
Description
Deconvolution of noisy data is an ill-posed problem, and requires some form of regularization to stabilize its solution. Tikhonov regularization is the most common method used, but it depends on the choice of a regularization parameter λ which must generally be estimated using one of several common methods. These methods can be computationally intensive, so I consider their behavior when only a portion of the sampled data is used. I show that the results of these methods converge as the sampling resolution increases, and use this to suggest a method of downsampling to estimate λ. I then present numerical results showing that this method can be feasible, and propose future avenues of inquiry.
Date Created
The date the item was original created (prior to any relationship with the ASU Digital Repositories.)
2015-05
Agent
- Author (aut): Hansen, Jakob Kristian
- Thesis director: Renaut, Rosemary
- Committee member: Cochran, Douglas
- Contributor (ctb): Barrett, The Honors College
- Contributor (ctb): School of Music
- Contributor (ctb): Economics Program in CLAS
- Contributor (ctb): School of Mathematical and Statistical Sciences